Your cart is currently empty!
SayPro Reviewing the effectiveness of 100-topic extraction per prompt, and proposing ways to improve the process.
SayPro is a Global Solutions Provider working with Individuals, Governments, Corporate Businesses, Municipalities, International Institutions. SayPro works across various Industries, Sectors providing wide range of solutions.
Email: info@saypro.online Call/WhatsApp: Use Chat Button 👇
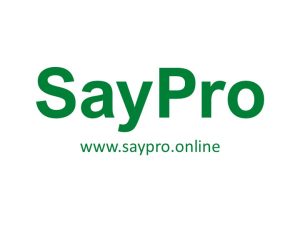
SayPro: Reviewing the Effectiveness of 100-Topic Extraction Per Prompt and Proposing Ways to Improve the Process
Introduction
SayPro utilizes advanced natural language processing (NLP) technology to extract relevant topics from textual data, with the goal of helping users gain insights, categorize content, and enhance content analysis. The process of extracting 100 topics per prompt, while ambitious, aims to provide comprehensive coverage of the text’s subject matter. However, the effectiveness of extracting such a large number of topics is contingent upon several factors, including accuracy, relevance, and efficiency. This report reviews the current approach to extracting 100 topics per prompt, evaluates its effectiveness, and proposes strategies for refining the process.
1. Current Topic Extraction Process: 100 Topics Per Prompt
The extraction of 100 topics per prompt is a challenging task that requires the AI to identify and categorize a large volume of information in a way that is both comprehensive and accurate. Typically, a prompt of this nature would contain large volumes of text or very complex data, necessitating precise extraction to ensure that the model captures key themes without overwhelming the user with irrelevant or redundant topics.
1.1 Strengths of the 100-Topic Extraction Approach
- Comprehensive Coverage: The primary strength of extracting 100 topics is the potential to provide a broad overview of the text, ensuring that even niche and less prominent themes are captured. This is particularly useful for research purposes or in situations where the entire range of topics is of interest.
- Depth of Insight: A larger set of extracted topics may uncover insights that would otherwise be missed in a smaller set, giving users more granular data to work with, especially when dealing with multi-faceted or highly detailed content.
- Flexibility in Application: For certain industries, such as legal or academic research, extracting 100 topics allows for thorough indexing, enabling users to search and filter by specific, highly detailed subjects within the text.
1.2 Challenges of Extracting 100 Topics Per Prompt
- Relevance and Precision: One of the primary concerns with extracting 100 topics is ensuring that they are all relevant to the context of the text. In an attempt to cover every possible theme, the model may extract topics that are either tangential or too generic, leading to less meaningful results for users.
- Redundancy: Given the sheer number of topics, there is a risk that the topics extracted may overlap or be too similar to one another. This redundancy dilutes the value of the output and requires additional manual effort to refine the results.
- User Overload: Presenting users with 100 topics in a single prompt can be overwhelming and counterproductive, especially if the user is seeking actionable insights. A list of 100 topics may be difficult to navigate and act upon effectively.
- Processing Complexity: Extracting and organizing 100 topics requires significant computational resources. The process may lead to longer processing times, higher server costs, and potential performance bottlenecks, especially when dealing with large volumes of text.
2. Evaluating the Effectiveness of the 100-Topic Extraction Approach
To assess the effectiveness of extracting 100 topics, several factors must be considered:
2.1 Quality of Extracted Topics
- Topic Relevance: Are the extracted topics truly reflective of the main themes in the text, or do they include tangential or irrelevant topics? The relevance of each topic is critical for users to draw meaningful conclusions.
- Specificity vs. Generalization: Does the model extract highly specific topics, or does it lean toward more general, broad categories? Specific topics are more actionable and valuable to users, while general topics may offer less utility.
- Redundancy and Diversity: How often do topics overlap or repeat? A high level of redundancy can reduce the value of having 100 topics, while a lack of diversity may indicate that the model is not fully capturing the breadth of the text’s content.
2.2 User Experience and Utility
- Ease of Use: Are users able to effectively navigate and utilize the 100 topics? In most cases, a large number of topics may overwhelm users rather than empower them. It is crucial to understand whether users are able to easily filter and extract insights from the long list of topics.
- Actionability: Do users find the topics actionable, or do they need further refinement and categorization? If the topics are too general or vague, users may struggle to apply them to real-world use cases.
- Efficiency: How quickly is the model able to extract and present 100 topics? Delays in processing time due to the large number of topics could lead to user frustration, especially for those who need rapid insights.
2.3 Computational Efficiency
- Processing Time: The time it takes to extract and categorize 100 topics per prompt is a key consideration. Extended processing times can negatively impact the user experience, particularly when dealing with large-scale data or high-frequency queries.
- Resource Allocation: The computational resources required to process a large number of topics may lead to higher costs. This can strain server capabilities, especially for businesses that scale quickly or handle significant volumes of data.
3. Proposed Strategies to Improve the 100-Topic Extraction Process
To address the challenges and optimize the extraction of 100 topics, the following strategies are recommended:
3.1 Refine the Model for Topic Relevance and Precision
- Enhanced Filtering: Introduce a more robust filtering mechanism that ensures extracted topics are highly relevant to the overall context of the text. The model could prioritize the extraction of topics that appear frequently or are semantically central to the text, reducing the inclusion of irrelevant or peripheral topics.
- Contextual Awareness: Enhance the model’s ability to differentiate between core and peripheral topics by improving its contextual awareness. This could involve fine-tuning the model to understand the document’s structure, whether it’s an academic paper, legal document, or customer feedback, to ensure that the extracted topics reflect the text’s intent.
- Eliminate Redundancy: Implement algorithms to identify and merge redundant or highly similar topics, presenting a diverse and non-overlapping list of topics. Techniques like clustering and synonym detection can be applied to group related topics together and remove duplicates.
3.2 Prioritize Actionable and User-Centric Topics
- Topic Grouping and Categorization: Instead of presenting all 100 topics at once, group related topics into broader categories. This would reduce the cognitive load for the user, allowing them to explore topics within structured categories such as “Environmental Issues,” “Technology Trends,” or “Health and Wellness.”
- User-Centric Filtering: Allow users to customize the topic extraction process based on their preferences. For example, users could specify the number of topics they wish to extract (e.g., top 10, top 20) or define the level of specificity they require. This flexibility can improve user satisfaction and make the process more actionable.
3.3 Optimize the Computational Process
- Batch Processing: Implement batch processing techniques to extract topics in smaller, manageable chunks, ensuring faster and more efficient processing. This would reduce the load on servers and decrease response time for users.
- Efficient Resource Allocation: Use AI models optimized for faster inference, especially when processing long texts or large datasets. Additionally, consider leveraging distributed computing for scalability to ensure faster performance during peak demand.
3.4 Provide Post-Extraction Tools for Topic Refinement
- Interactive Topic Refinement: Once the 100 topics are extracted, provide users with the ability to refine and modify the topics through an interactive interface. This could include options for removing irrelevant topics, combining similar ones, or manually tagging topics for further categorization.
- Topic Validation: Include a mechanism for users to validate the extracted topics by allowing them to rate the relevance of each topic. This feedback can be used to fine-tune the extraction model and continuously improve its performance.
3.5 Reduce the Number of Topics for Usability
- Topic Limitations: Instead of extracting 100 topics, consider reducing the number to a more manageable number, such as 20 or 30, and presenting the most important and relevant ones. For highly complex texts, prioritize diversity and specificity over sheer volume, ensuring that the topics extracted truly represent the most significant themes in the text.
4. Conclusion
While extracting 100 topics per prompt has the potential to provide a comprehensive overview of a text, it comes with significant challenges related to relevance, redundancy, user experience, and computational efficiency. By refining the extraction process to prioritize meaningful, actionable topics and optimizing the computational backend, SayPro can enhance its topic extraction capabilities, providing more value to users. Adopting the strategies outlined in this report will lead to more efficient, relevant, and user-friendly topic extraction, ultimately improving the quality of insights provided to clients and boosting overall satisfaction.
Leave a Reply