Your cart is currently empty!
SayPro Fundraising Data Cleanup: Ensuring Accuracy and Integrity.
SayPro is a Global Solutions Provider working with Individuals, Governments, Corporate Businesses, Municipalities, International Institutions. SayPro works across various Industries, Sectors providing wide range of solutions.
Email: info@saypro.online Call/WhatsApp: Use Chat Button 👇
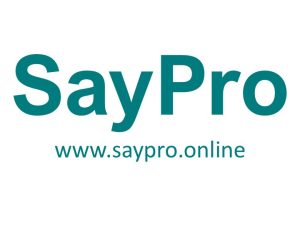
Data cleanup is a critical process in SayPro’s fundraising efforts, ensuring that the information collected from various sources is accurate, consistent, and reliable. In any fundraising organization, raw data is collected from multiple channels, such as online donations, social media interactions, event registrations, and offline pledges. However, this data often contains inconsistencies, duplicates, errors, or even missing values. Data cleanup aims to correct these issues, transforming the raw data into a structured and usable format, thus enhancing the accuracy of the analysis and insights derived from it.
1. Importance of Data Cleanup in Fundraising
Effective data cleanup is essential for the following reasons:
- Accurate Reporting: Clean and organized data ensures that fundraising performance reports, donor demographics, and campaign analytics are based on solid foundations, providing the organization with actionable insights.
- Improved Donor Relationships: Clean data ensures that communications, acknowledgments, and follow-ups with donors are personalized, relevant, and timely. Inaccurate or inconsistent data can lead to missed opportunities or miscommunication, which could harm donor relationships.
- Compliance and Security: For organizations like SayPro, adhering to data protection regulations (e.g., GDPR or CCPA) is crucial. Data cleanup helps remove unnecessary data and ensures that the remaining information is properly stored and handled according to legal requirements.
- Enhanced Decision-Making: Clean data facilitates better decision-making, as it provides reliable insights into donor behavior, campaign effectiveness, and fundraising outcomes.
2. Key Steps in Data Cleanup
The data cleanup process for SayPro involves multiple steps, each designed to address different aspects of the data quality issues. Here’s a breakdown of the steps involved:
a. Removing Duplicates
One of the most common issues in fundraising data is duplicate entries, where the same donor or transaction is recorded multiple times. This can happen due to different data entry points (e.g., online and offline channels) or errors during data collection.
Steps to remove duplicates:
- Identification: Use data analysis tools to find duplicate records. This could involve matching key fields like name, email address, phone number, or donor ID.
- Deduplication Process: Automatically or manually merge duplicate records into a single entry, ensuring that all information is consolidated (e.g., combining donation history from different records into one).
- Preventative Measures: Implement data validation checks at the point of entry to prevent future duplication (e.g., using unique donor IDs or email address matching during online registration).
b. Correcting Errors
Data errors can arise from various sources, such as human input mistakes, system glitches, or incorrect data mappings. These errors can severely impact the accuracy of analysis and decision-making.
Steps to correct errors:
- Spotting Inconsistencies: Identify common errors in the dataset, such as misspelled names, incorrect donor information (e.g., wrong addresses or email formats), or numerical inconsistencies (e.g., invalid donation amounts or missing values).
- Standardization: Standardize the format of common data fields. For example, ensure that names are entered consistently (e.g., first name followed by last name, no initials), email addresses are in a uniform format (lowercase), and phone numbers follow a standard format.
- Data Validation: Implement validation checks on data entry forms (e.g., using drop-down menus for states or countries, validating email formats, or using date pickers for date entries) to prevent incorrect entries.
c. Standardizing Entries
Consistency in data formatting and terminology is essential to ensure that analysis can be carried out effectively. Without standardization, it becomes difficult to aggregate and compare data across different records.
Steps to standardize entries:
- Categorical Standardization: Ensure that categorical data (e.g., donor type, event participation, payment methods) uses consistent terminology. For instance, if some records use “Credit Card” and others use “Creditcard,” these should be standardized to a single term (e.g., “Credit Card”).
- Date Format Standardization: Dates should be entered in a uniform format (e.g., MM/DD/YYYY or DD/MM/YYYY) to avoid confusion when performing time-based analysis.
- Address Formatting: Standardize the address formatting to ensure consistency in reporting and mailing. For example, use consistent abbreviations (e.g., “Street” vs. “St.”) and always include the full postal code.
d. Handling Missing Data
Missing data can be a significant issue in any dataset, leading to gaps in donor records, donation amounts, or event participation. In fundraising, missing data can skew the analysis and lead to faulty conclusions.
Steps to handle missing data:
- Identifying Missing Data: Run reports to identify missing or incomplete fields, such as missing email addresses, donation amounts, or contact details.
- Imputation or Replacement: If possible, impute missing data by using statistical techniques or business rules (e.g., filling in missing donation amounts with averages or estimates). Alternatively, use common sense to replace missing values (e.g., reach out to the donor for missing contact information).
- Removing Records: If a record has critical missing information that cannot be recovered or estimated, consider removing it from the database to avoid skewing the results.
e. Normalizing Data Across Different Sources
When data is collected from multiple channels (e.g., online donations, social media, events, offline donations), it may follow different formats or conventions. It is important to normalize this data to ensure consistency across all sources.
Steps for normalization:
- Field Mapping: Map fields from different systems to ensure that the same information is captured in a consistent way (e.g., “First Name” in one system may be called “Donor Name” in another; these should be aligned).
- Source Consolidation: If SayPro collects data through various platforms, ensure that each piece of data is tied back to the same donor record. This might include linking online and offline donations to a single donor profile.
- Currency and Amount Standardization: If donations are recorded in different currencies or formats (e.g., “$100” vs. “100 USD”), normalize these into a single currency and format to avoid discrepancies in financial reports.
f. Validating Data Quality
Before using the cleaned data for analysis or reporting, it’s essential to validate its quality. This step involves reviewing the cleaned data to ensure it meets the required standards for completeness, accuracy, and consistency.
Steps for validation:
- Automated Checks: Use automated tools to perform data integrity checks (e.g., ensuring that there are no negative donation amounts, invalid donor emails, or empty fields).
- Spot Checking: Conduct manual spot checks on random records to ensure that the data cleanup process has been applied correctly.
- Consistency Checks: Cross-check cleaned data against original source data (e.g., donor confirmation emails or registration forms) to ensure that no key information has been lost during the cleanup process.
3. Ongoing Data Maintenance
Data cleanup is not a one-time task; it should be an ongoing process. As SayPro continues to collect data through various fundraising campaigns, events, and donor interactions, it’s essential to maintain a routine for cleaning and updating the database.
Ongoing data maintenance practices:
- Regular Audits: Conduct periodic data audits to ensure data integrity over time and address any issues that arise as the dataset grows.
- Continuous Validation: Regularly update and refine validation rules to reflect new data collection practices, emerging trends, and changes in the organization’s operations.
- Training and Best Practices: Ensure that team members involved in data entry and collection are trained on data standards and best practices to reduce errors and inconsistencies.
4. Conclusion
Data cleanup is an essential part of SayPro’s fundraising data management. By removing duplicates, correcting errors, standardizing entries, handling missing data, and normalizing data from multiple sources, SayPro can ensure that its data is accurate, consistent, and reliable. This ultimately allows for better insights, improved donor relations, and more effective decision-making, all of which contribute to the success of fundraising campaigns and organizational growth.
Leave a Reply