Your cart is currently empty!
SayPro Data Entry and Quality Assurance
SayPro is a Global Solutions Provider working with Individuals, Governments, Corporate Businesses, Municipalities, International Institutions. SayPro works across various Industries, Sectors providing wide range of solutions.
Email: info@saypro.online Call/WhatsApp: Use Chat Button π
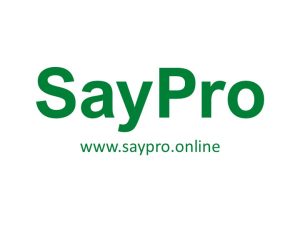
Key Responsibility: Data Entry and Quality Assurance
- Monitor Data Entry Process:
- Oversee the data entry process, ensuring all collected data is entered into the system accurately and consistently.
- Implement real-time data validation checks during entry to detect errors immediately, such as missing values or inconsistencies.
- Assign data entry tasks to trained personnel or automated systems, ensuring all individuals involved follow the correct procedures.
- Ensure data is entered in the appropriate format to maintain consistency and alignment with the research methodology.
- Quality Assurance and Accuracy Checks:
- Conduct regular quality assurance reviews to verify that entered data matches the original sources.
- Perform spot-checks and cross-validation by comparing entries with source documents (e.g., survey responses, interview transcripts) to identify and correct discrepancies.
- Utilize automated data entry software or tools to reduce human error and ensure a higher level of accuracy in the data collection process.
- Develop and implement a Data Quality Assurance Report, documenting any issues encountered during the entry process, along with corrective actions taken.
- Develop Data Quality Control Protocols:
- Establish clear protocols for handling common data entry issues, such as duplicates, invalid responses, or inconsistent formats.
- Set up clear guidelines for data entry personnel, ensuring they adhere to the best practices for capturing and entering data without errors.
- Ensure there is an ongoing training program for staff involved in data entry, emphasizing the importance of accuracy and attention to detail.
- Track and Address Data Discrepancies:
- Implement a system for tracking discrepancies in the data during entry and ensure these issues are reported and addressed immediately.
- Work with field teams to clarify missing or unclear data before data entry is finalized, ensuring that incomplete records are flagged for resolution.
- Review and Finalize Data Quality:
- At the end of each data collection cycle, ensure the collected data is cleaned, categorized, and formatted for analysis.
- Validate that the final dataset is free from duplicate entries, errors, and outliers that may affect the results of the study.
- Collaborate with the analysis team to confirm the data is ready for the next stages of analysis, ensuring all quality assurance processes are documented and met.
- stablish Data Integrity Protocols:
- Develop and implement data integrity protocols that define the process for checking and maintaining the accuracy, consistency, and reliability of the data throughout the collection and entry phases.
- Ensure all team members involved in data handling understand the importance of data integrity and follow established standards to protect it.
- Conduct Real-Time Data Quality Checks:
- Implement real-time checks during data entry to immediately detect and correct errors such as missing fields, inconsistent formatting, and duplicate entries.
- Use automated validation tools where possible to flag discrepancies or outliers as data is entered into the system.
- Ensure that data is entered according to the predefined data collection templates to maintain consistency and reduce human error.
- Periodic Review and Auditing of Data:
- Conduct periodic audits to review data at various stages of collection and entry.
- Spot-check data regularly by comparing entered data with original sources (e.g., survey forms, interview transcripts) to identify any discrepancies or potential issues with the entered information.
- Use data consistency checks to verify that different datasets align and are correctly formatted for easy analysis.
- Address Data Integrity Issues Promptly:
- Implement a system to track and log data discrepancies, allowing for efficient identification and correction.
- Set up clear communication channels with data entry personnel and field teams to quickly resolve any issues affecting data quality.
- Address inconsistencies immediately, ensuring that corrective actions are taken before the data is used for analysis or reporting.
- Data Integrity Testing and Verification:
- Conduct integrity testing to ensure that datasets have not been altered, lost, or corrupted during the data collection and entry process.
- Validate data integrity at multiple stages, including during the initial entry, upon completion of the collection process, and before final analysis.
- Use data profiling tools to ensure the data conforms to expected patterns, checking for issues like missing values, duplicate records, or outlier results.
- Final Quality Assurance Review:
- Before the data is submitted for analysis, perform a final quality check to ensure all collected data is cleaned, categorized, and formatted appropriately.
- Verify that no errors or inconsistencies remain, and ensure that the dataset is ready for analysis.
- Generate a Data Quality Assurance Report documenting the steps taken to ensure data integrity and any issues that were addressed during the process.
Leave a Reply