Your cart is currently empty!
SayPro Data Cleansing and Standardization Process.
SayPro is a Global Solutions Provider working with Individuals, Governments, Corporate Businesses, Municipalities, International Institutions. SayPro works across various Industries, Sectors providing wide range of solutions.
Email: info@saypro.online Call/WhatsApp: Use Chat Button 👇
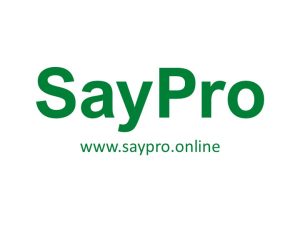
Objective: The goal of this phase is to cleanse and standardize the fundraising data to ensure that it is accurate, consistent, and ready for analysis and decision-making. Data cleansing and standardization is a critical step in ensuring the integrity of the information, which will be used for insights into donor behavior, campaign success, and more. By standardizing and cleaning the data, SayPro can ensure that no errors, duplicates, or inconsistencies affect the quality of reports, analyses, and outreach efforts.
Step-by-Step Process for Data Cleansing and Standardization
1. Identifying Common Data Issues
Before proceeding with the cleansing and standardization, it’s important to identify common issues in the collected data. These issues may include:
- Duplicate Entries: The same donor may appear multiple times, either due to slight variations in their data (e.g., different spellings of their name or different contact details).
- Inconsistent Data Formats: Data may be recorded in different formats across sources (e.g., dates in different styles, inconsistent capitalization of names or addresses).
- Missing Values: Some donor information (like email addresses or donation amounts) may be missing or incomplete.
- Erroneous Data: Errors may have occurred during data entry, such as incorrect donation amounts or misspelled names.
- Invalid Data: Data that is simply incorrect or does not fit the required format, such as an invalid email address or phone number, should be flagged for review.
2. Standardizing Data Formats
Once the data issues are identified, the next step is to standardize the data so it follows a consistent structure. This will make it easier to analyze and will reduce errors in future processing. Common standardization procedures include:
- Date Formatting: Dates should be standardized to a single format across all data entries. For instance, use the MM/DD/YYYY format or YYYY-MM-DD. Ensure that dates like “January 5, 2025” are consistently converted to one format.
- Example: “01/05/2025” or “2025-01-05” depending on the chosen format.
- Name Formatting: Ensure all names are capitalized consistently. If you’re dealing with full names, make sure each word in the name is properly capitalized (e.g., “john doe” should become “John Doe”).
- Example: “john smith” → “John Smith”
- Address Standardization: Standardize addresses by ensuring consistency in street types (e.g., “St.” vs. “Street”), abbreviations (e.g., “Ave.” vs. “Avenue”), and the full use of city and state names. This may include adding missing zip codes or postal codes if they’re incomplete.
- Example: “123 main st.” → “123 Main St.”
- Phone Numbers: Standardize phone numbers to a consistent format (e.g., (XXX) XXX-XXXX). Ensure all international phone numbers are included in a recognized international format, with country codes if applicable.
- Currency Formatting: Ensure all donation amounts are recorded with a consistent currency format, including the correct decimal places and symbols (e.g., USD, €, etc.). If necessary, remove any symbols or text that do not represent monetary values.
- Email Validation: Standardize email formats by ensuring they follow the correct structure (e.g., user@domain.com). Use regular expressions or email validation tools to check if the emails are correctly formatted.
3. Removing Duplicates
Duplicate entries in the dataset can severely impact the accuracy of reports and analysis. To cleanse the data, you need to:
- Identifying Duplicates: Identify duplicate records based on key attributes like:
- Full name
- Email address
- Phone number
- Donation amount (with the same date)
- Consolidating Duplicate Entries: If multiple entries exist for the same donor, merge them into a single record, combining donation history and any other associated information. You might need to combine multiple donation amounts or contact details if they appear under different entries.
- Automatic Deduplication Tools: Utilize deduplication tools or algorithms, such as Excel’s “Remove Duplicates” feature or data cleansing software like OpenRefine, Talend, or Python-based solutions using libraries like pandas.
- Reviewing and Approving: In cases of minor discrepancies (e.g., slight variations in the donor’s name or email), consider reviewing the records manually to ensure you aren’t merging distinct individuals by mistake.
4. Handling Missing Data
Missing data is a common issue in fundraising datasets and needs to be handled carefully:
- Identifying Missing Data: Identify and flag missing values in key fields, such as name, email, donation amount, and donation date. These fields may be empty or contain placeholders like “TBD” or “Unknown.”
- Filling in Missing Data:
- Contacting Donors: If possible, reach out to the donors who provided incomplete information to request the missing details (e.g., email addresses or donation amounts).
- Using Default Values: For non-critical fields, you can use default placeholders, such as “N/A” for missing phone numbers.
- Excluding Incomplete Records: In some cases, it may be best to exclude records with critical missing information, especially if those records cannot be validated or completed.
- Imputation Techniques: If your dataset is large and missing data is a concern, you can use imputation techniques (e.g., filling in missing donation amounts based on averages or trends) to make educated guesses about missing values.
5. Removing Erroneous Data
Erroneous data is any incorrect or nonsensical data that could skew analysis. For example:
- Donation Amounts: Check that donation amounts are realistic and consistent. Donations listed as $0 or negative amounts should be reviewed or excluded. If a donor accidentally enters “$5000” when they meant to donate $50, this error must be fixed.
- Invalid Emails: Identify and remove or correct any invalid email addresses that do not follow the correct format or are flagged as incorrect (e.g., emails with missing domains or typo errors).
- Address Validation: Use address validation tools to check if the provided addresses are valid or if they point to nonexistent locations.
6. Validating and Testing the Cleaned Data
After cleansing the data, it is crucial to validate and test its accuracy and completeness:
- Automated Validation Tools: Use data validation software to check for any remaining inconsistencies, missing values, or errors. For example, use a script to automatically check for out-of-range values, or use data validation formulas in Excel to highlight discrepancies.
- Spot Checks: Perform random sampling on various sections of the dataset to manually check the integrity of the data. This could include verifying a donor’s donation history, checking the accuracy of email addresses, or confirming the correctness of a donor’s contact details.
- Cross-Referencing: Compare the cleaned dataset with original source documents or other reliable records to ensure that all data points match and are accurate.
7. Final Review and Documentation
Once the data has been cleansed and standardized:
- Final Review: Conduct a final review to ensure that the data is consistent, accurate, and ready for use in reporting or analysis. This is the last opportunity to catch any lingering issues before the data is used in decision-making.
- Documentation: Document the data cleaning process, outlining the steps taken, the tools used, and any specific assumptions made during the process. This documentation will be helpful in future projects and in maintaining data integrity over time.
Outcome of the Data Cleansing and Standardization Process
After completing the cleansing and standardization process, SayPro will have:
- Accurate and Consistent Data: The data will be free of duplicates, errors, and inconsistencies.
- Standardized Formats: All data will follow consistent formats for dates, names, addresses, and donation amounts, making it easier to analyze.
- Valid and Complete Records: Missing or erroneous data will be addressed, leaving behind only valid, complete records.
- Ready-to-Use Dataset: The data will be ready for analysis, reporting, and decision-making, ensuring that the fundraising efforts can be accurately assessed.
This meticulous approach to cleansing and standardizing the data will provide a solid foundation for future fundraising campaigns, donor analysis, and reporting.
Leave a Reply