Your cart is currently empty!
SayPro Analyzing the topic extraction efficiency over January 2025 using SayPro’s platform. Identifying key areas where GPT prompts generated the most useful topics.
SayPro is a Global Solutions Provider working with Individuals, Governments, Corporate Businesses, Municipalities, International Institutions. SayPro works across various Industries, Sectors providing wide range of solutions.
Email: info@saypro.online Call/WhatsApp: Use Chat Button 👇
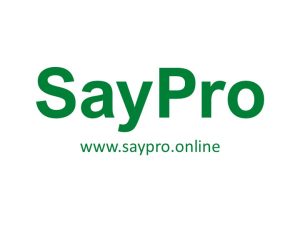
SayPro: Analyzing Topic Extraction Efficiency Over January 2025 Using SayPro’s Platform
At SayPro, evaluating the efficiency of topic extraction is crucial for ensuring that the GPT-based systems are effectively delivering relevant, actionable insights. By analyzing the performance of topic extraction over a specific time frame, such as January 2025, SayPro can assess the accuracy of the GPT prompts, track areas of improvement, and identify key areas where the system has been most beneficial. This analysis helps to fine-tune the system, optimize its output, and ensure that the data generated aligns with business goals.
Here is a detailed approach to analyzing topic extraction efficiency using SayPro’s platform in January 2025, including identifying key areas where GPT-generated topics were most useful:
1. Data Collection for Topic Extraction
Before analyzing the efficiency of GPT-generated topics, it’s essential to gather the data generated by the topic extraction process. SayPro likely uses a structured data pipeline that pulls in text sources such as documents, meeting notes, customer feedback, or product reports that are fed into the GPT model for topic extraction.
Steps in Data Collection:
- Input Data Sources: Identify the text sources used in topic extraction. These could include internal documents (e.g., reports, memos), customer interactions (e.g., chat logs, emails), or knowledge bases (e.g., FAQs, articles).
- GPT Prompts and Outputs: Track the prompts used by the GPT model to extract topics from the data. Each prompt will generate a set of topics, which will be analyzed for their relevance and usefulness.
- Timestamping Data: Ensure that all topic extraction data is timestamped to track performance over the month of January 2025, allowing for temporal comparisons.
- Categorization: Group the topics based on the type of data source. For example, customer feedback topics, internal document topics, or external research topics. This segmentation can help identify which sources provide more valuable results.
2. Measuring Efficiency of Topic Extraction
Once data is collected, the next step is to evaluate the efficiency of topic extraction over January 2025. Efficiency can be measured in terms of both accuracy (how relevant and specific the extracted topics are) and processing speed (how quickly the GPT model generates the topics).
Key Efficiency Metrics:
- Topic Relevance: Evaluate how well the extracted topics match the content of the source materials. For example, if GPT extracts the topic “product feedback” from a set of customer reviews, how closely does this topic relate to the issues discussed in the reviews?
- Metric: The percentage of relevant topics (i.e., topics that are deemed useful based on a manual review or user feedback).
- Topic Specificity: Assess how specific or generalized the topics are. For example, broad topics like “customer satisfaction” might be less useful than more specific ones like “customer dissatisfaction with delivery time.”
- Metric: Number of specific topics versus general topics.
- Processing Time: Track how long it takes the GPT model to process the input data and extract topics. Efficiency is also about getting timely results to support decision-making.
- Metric: Average time to process a document and generate topics.
- Accuracy of Extraction: Manually review a sample of the extracted topics to determine how accurate they are in relation to the data. This could involve a comparison between the GPT output and human-generated topics.
- Metric: Percentage of correctly identified topics compared to human-assigned topics (e.g., 95% of topics matched human labels).
- User Feedback: Analyze feedback from internal teams (e.g., content creators, customer support) who use the extracted topics. Do they find the topics useful? Do they use the generated topics in their work? Gathering direct feedback helps refine the system and assess its usefulness.
- Metric: Satisfaction ratings or qualitative feedback from teams using the extracted topics.
3. Identifying Key Areas of High Efficiency
After gathering and measuring the data, the next step is to identify which areas and types of GPT prompts have yielded the most useful topics during January 2025. Key areas of high efficiency may vary depending on the source data, the complexity of the prompt, and the purpose of the topic extraction.
Key Areas of Focus for Topic Extraction:
- Customer Feedback:
- Use Case: One of the most critical areas for GPT topic extraction is customer feedback. GPT can generate actionable topics that help teams address recurring customer concerns or identify popular feature requests.
- Key Finding: During January 2025, GPT may have been particularly efficient in extracting topics related to product issues (e.g., “slow shipping times” or “difficult return process”), which could help customer support teams focus their efforts on resolving frequent pain points.
- Example: If the GPT prompt focused on extracting common complaints from customer emails or surveys, topics like “shipping delays,” “product defects,” and “customer service response times” may have been highly relevant and actionable.
- Internal Document Review:
- Use Case: For internal documents such as team reports or project updates, GPT can help identify key insights or trends that may be buried in lengthy documents.
- Key Finding: In this area, GPT could have been especially effective in extracting high-level topics related to performance metrics (e.g., “sales growth,” “employee productivity”) or project status updates (e.g., “delays in project X,” “milestone achieved”).
- Example: For a product development team, GPT may have been able to identify relevant topics like “feature development progress” or “testing feedback,” which can help in prioritizing the next steps for the team.
- Industry Research:
- Use Case: For market research or competitive analysis reports, GPT can generate topics that help identify key trends or emerging opportunities.
- Key Finding: In January 2025, GPT may have been particularly effective in extracting market trends (e.g., “AI adoption,” “remote work solutions”) from industry reports or news articles, providing insights that were beneficial for strategic decision-making.
- Example: GPT might have efficiently extracted topics like “growth in e-commerce” or “shift toward cloud computing,” which could be critical for SayPro’s market positioning.
- Knowledge Base and FAQ Documents:
- Use Case: A large knowledge base or frequently asked questions (FAQ) document may contain valuable information that can be synthesized into key topics for customers or employees.
- Key Finding: GPT could have generated support-related topics (e.g., “account management,” “billing inquiries”) that can help streamline customer interactions and improve self-service options for users.
- Example: For a customer service team, extracting topics like “password recovery,” “account updates,” or “payment issues” would allow them to address the most common inquiries with tailored solutions.
4. Challenges and Areas for Improvement
While GPT’s topic extraction capabilities are useful, there may also be challenges and areas where improvement is needed. Some of the potential areas for refinement in January 2025 include:
- Ambiguity in Topics: Sometimes GPT can generate overly broad or ambiguous topics that aren’t actionable. For example, a topic like “user experience” might be too vague without further breakdown.
- Improvement: Fine-tune the prompts to extract more specific topics, such as “user experience in mobile app navigation” or “customer satisfaction with checkout process.”
- Inconsistent Extraction Across Sources: GPT may perform better with certain data types (e.g., customer feedback) and less well with others (e.g., technical reports).
- Improvement: Implement better contextual understanding in the GPT model by customizing the model for specific data sources or use cases.
- Performance Monitoring and Adjustment: Over time, the GPT model’s performance needs to be continuously monitored to ensure it’s improving. If certain prompts aren’t yielding useful topics, adjustments should be made.
- Improvement: Implement a feedback loop where teams can rate the relevance of extracted topics, allowing continuous training and fine-tuning of the GPT model.
5. Actionable Insights and Recommendations
Based on the analysis, SayPro can develop actionable recommendations to enhance the GPT-based topic extraction system:
- Optimize Prompts: Continue refining GPT prompts to increase the specificity and relevance of the topics extracted.
- Focus on Key Areas: Encourage teams to provide feedback on which types of documents and data sets produce the most valuable topics, focusing future efforts on these areas.
- Continuous Model Training: Regularly update and retrain the GPT model based on feedback and observed performance over time.
- Automate Follow-Up Actions: Based on extracted topics, automate actions for teams (e.g., creating follow-up tasks or alerts when a certain topic is identified, like “product defect”).
Conclusion
By analyzing GPT topic extraction efficiency over January 2025, SayPro can identify areas where the system excels and areas where it requires improvement. Focusing on key areas of high efficiency, such as customer feedback and internal documents, while addressing challenges like topic ambiguity and inconsistency, will enable SayPro to continuously refine its topic extraction processes. This ongoing improvement ensures that SayPro’s teams are always working with the most relevant, actionable insights.
Leave a Reply