Your cart is currently empty!
Author: Andries Makwakwa
SayPro is a Global Solutions Provider working with Individuals, Governments, Corporate Businesses, Municipalities, International Institutions. SayPro works across various Industries, Sectors providing wide range of solutions.
Email: info@saypro.online Call/WhatsApp: Use Chat Button 👇
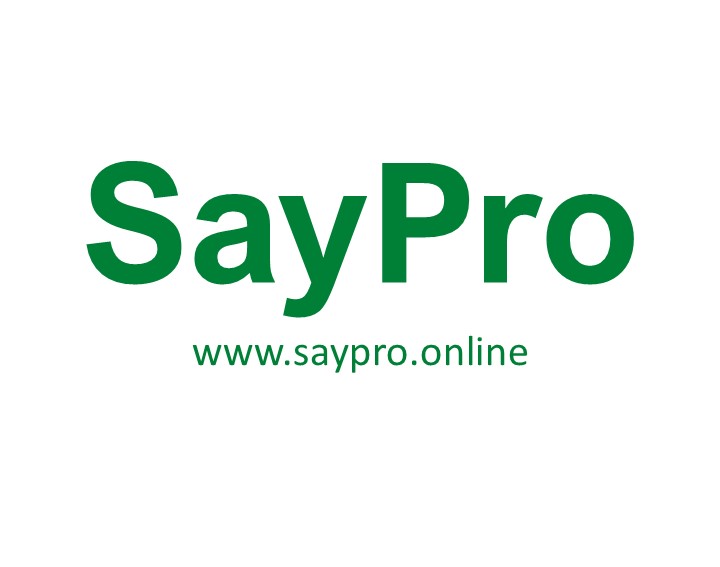
SayPro Data Collection: Gathering all necessary information from various teams within SayPro, including task completion rates, document management statistics, and results from GPT-based topic extractions.
SayPro Data Collection: A Detailed Overview
Data collection is a crucial process for understanding the performance, challenges, and opportunities within any business or organization. For SayPro, a company utilizing advanced tools like GPT for topic extraction and working across various departments, gathering the necessary data involves systematically pulling together relevant metrics, results, and statistics from various teams to make data-driven decisions. Here’s how this process unfolds in detail:
1. Task Completion Rates
Task completion rates are a key performance indicator (KPI) that measure the efficiency of different teams within SayPro. This data reflects the proportion of tasks completed versus those assigned, providing insight into how well teams are managing workloads.
Data Collection Process:
- Team Inputs: Each team within SayPro submits reports detailing tasks that were assigned, in progress, and completed. These reports should include the task description, the assigned team member, the date of assignment, and the completion date.
- Task Tracking Tools: SayPro likely uses task management tools (e.g., Asana, Jira, Trello) where tasks are categorized, assigned, and tracked. These tools can provide automated reports on the number of tasks completed within a given period and track progress against deadlines.
- Time Analysis: The data collected from these tools will also include time spent on each task. This helps in calculating efficiency and understanding whether tasks are being completed within the expected timelines.
- Quality Metrics: In addition to completion rates, qualitative assessments of task quality may be gathered through team feedback or post-task evaluations to ensure that completed tasks meet the company’s standards.
Key Metrics:
- Total number of tasks assigned
- Number of tasks completed
- Task completion rate (%) = (Number of tasks completed / Total number of tasks assigned) * 100
- Average completion time per task
- Team performance analysis (e.g., team A completed 95% of its tasks on time, while team B completed 80%)
2. Document Management Statistics
Document management is an essential aspect of SayPro’s operations, especially if the company handles significant amounts of information. Accurate management of documents ensures easy access, security, and compliance with regulations.
Data Collection Process:
- Document Tracking Systems: SayPro likely uses document management systems (DMS) such as SharePoint, Google Workspace, or proprietary systems. These platforms can track the creation, modification, sharing, and archiving of documents.
- Document Upload and Access Rates: Data should be gathered on the number of documents uploaded, edited, and accessed over time. This gives insight into the volume of work being handled, as well as which documents are most frequently accessed or in demand.
- Version Control and Collaboration: Collect data on document revisions and edits. How many versions of a document were created and what percentage of documents were co-authored or commented on by multiple team members? This is critical for understanding collaboration patterns within teams.
- Compliance and Security: Track whether documents comply with internal and external regulations (e.g., GDPR for personal data), and whether they are being stored and accessed securely. Security logs from the DMS system can provide information about unauthorized access attempts or document retrieval issues.
Key Metrics:
- Number of documents uploaded
- Frequency of document access and edits
- Number of collaborative documents (documents edited by multiple users)
- Document version count (how often documents are updated)
- Document retrieval time (how quickly can a document be located and accessed)
- Security and compliance adherence metrics
3. GPT-Based Topic Extractions and Results
GPT (Generative Pretrained Transformer) models, such as the ones used by SayPro, help in extracting topics, summarizing documents, and providing insights from unstructured data. Data collected from GPT-based topic extraction will allow the company to evaluate how well the model is performing in various applications.
Data Collection Process:
- Model Input Data: Collect information on the input data provided to the GPT model. This includes text sources such as documents, chat logs, customer feedback, or knowledge base entries that are processed by the model.
- Topic Extraction Accuracy: Measure the effectiveness of the GPT model in identifying relevant topics. This can involve collecting user feedback from teams who use the extracted topics and categorizing whether the topics are useful and actionable.
- Data on Usage: Collect data on how often the GPT model’s results are used by different teams. This includes which departments are leveraging topic extraction results, how they integrate it into their workflows, and the tangible outcomes (e.g., better customer service, more efficient content creation).
- Error Rate and Refinements: Keep track of errors or misclassifications made by the GPT model. This could include cases where the model misunderstood a document’s main themes, leading to irrelevant or inaccurate topic suggestions. Also, monitor any ongoing model training or fine-tuning efforts.
- Turnaround Time: Collect data on how quickly the GPT model processes input data and generates topic extractions, as this will affect operational efficiency and user satisfaction.
Key Metrics:
- Accuracy of extracted topics (percentage of topics correctly identified)
- User satisfaction ratings (from teams using the extracted topics)
- Frequency of GPT model usage by different teams
- Error rates and types (misclassifications, irrelevant results)
- Average time taken for GPT to process and generate results
- Model improvements and training data feedback
4. Integrating Data Across Teams
Once data is collected from each team, it must be consolidated in a central system where it can be analyzed and used for decision-making. SayPro might use tools such as dashboards, spreadsheets, or business intelligence software (e.g., Power BI, Tableau) to integrate and visualize the data from task completion, document management, and GPT-based topic extraction.
Data Integration Process:
- Centralized Reporting System: All collected data from various teams should be routed into a centralized reporting system for analysis. This system can automatically aggregate data, identify trends, and visualize performance across the company.
- Team Collaboration and Feedback: Different teams need to provide feedback on the relevance and usefulness of the collected data. This will help refine data collection methods, reporting systems, and task assignment strategies.
Key Metrics for Data Integration:
- Consolidation speed (how quickly can data from all teams be processed and visualized)
- Cross-departmental performance analysis (how well different departments are meeting their KPIs)
- Feedback from teams on data relevance and usefulness
- Data accuracy and consistency across teams
Conclusion
The data collection process at SayPro involves detailed tracking across multiple areas—task completion, document management, and GPT-based topic extractions. By gathering comprehensive data from all relevant teams, SayPro can assess performance, identify areas for improvement, and ensure that advanced tools like GPT are being effectively leveraged for topic extraction. The collected data also allows the company to refine workflows, boost productivity, and improve decision-making processes across departments. This structured approach to data collection ensures that SayPro remains data-driven, efficient, and responsive to internal and external demands.
SayPro Collaboration with SayPro Departments: Communicating with external stakeholders if necessary to gather data that is beyond SayPro’s internal scope.
SayPro Collaboration with SayPro Departments: Communicating with External Stakeholders to Gather Data Beyond SayPro’s Internal Scope
At SayPro, successful data retrieval and effective data-driven decision-making are not limited to internal systems and repositories. Often, the data needed for comprehensive analysis and reporting may extend beyond the organization’s internal scope, requiring communication and collaboration with external stakeholders. These stakeholders could be suppliers, clients, third-party data providers, regulatory bodies, or industry partners that offer specialized data or insights critical to SayPro’s operations.
The collaboration process for obtaining data from external sources involves careful planning, coordination, and communication to ensure the accurate, timely, and legal gathering of data. This process requires collaboration not only between different departments within SayPro but also with external parties to ensure that the data retrieved meets business needs while maintaining compliance and quality standards.
Below is a detailed breakdown of how SayPro facilitates this collaboration with external stakeholders, outlines the necessary steps, and ensures that data is gathered efficiently and ethically.
1. Purpose of External Collaboration
External collaboration is critical for obtaining data that is either not available within SayPro’s internal systems or is better sourced from outside the organization. The purpose of this collaboration includes:
- Filling Data Gaps: Ensuring that data gaps in internal records are filled by obtaining relevant data from third-party vendors or external databases.
- Enhancing Analysis: Integrating external data to enrich internal datasets, enabling more comprehensive and insightful analysis (e.g., industry benchmarks, market trends).
- Ensuring Compliance: Acquiring regulatory, compliance, or legal data that might be necessary for audits, reports, or business operations.
- Data Enrichment: Complementing internal data with third-party data to gain additional context, such as demographic information, consumer behavior, or market intelligence.
- Broader Insights: Accessing external research, surveys, or reports that may provide a broader view of trends or performance indicators in the industry.
2. Key Steps in Collaborating with External Stakeholders
The process of communicating with and collaborating with external stakeholders is multifaceted, and SayPro must ensure that each step is carefully executed to gather accurate, relevant, and usable data. Below is a detailed description of the key steps involved:
a) Identify Data Needs and External Sources
The first step in the collaboration process is to identify the data needs and determine the external sources that can provide the necessary data. This involves:
- Clarifying Data Requirements: Understanding the specific data needed for the project, report, or analysis. For example, SayPro might need external data on industry trends, customer demographics, or competitor performance metrics.
- Mapping External Data Sources: Identifying potential external stakeholders who can provide the required data. These might include:
- Industry partners: Competitors, suppliers, and other stakeholders in the same industry.
- Government agencies: Regulatory bodies that provide industry or market data.
- Market research firms: Companies that specialize in gathering and analyzing market data.
- Consultants and vendors: External consultants who offer specialized datasets for purchase or subscription.
- Public or proprietary databases: Third-party providers who offer data, such as financial data, academic research, or customer behavior insights.
b) Evaluate the Feasibility of Data Sharing
Once the external sources have been identified, the next step is to evaluate whether the external data can be shared with SayPro. This evaluation includes:
- Data Availability: Assessing whether the required data is publicly available, accessible via a subscription, or requires permission to be shared.
- Data Format: Understanding the format of the data (e.g., CSV, API, database access) and determining if it can be integrated into SayPro’s internal systems or analysis tools.
- Data Quality: Ensuring that the external data meets the quality standards that SayPro needs for reliable analysis, including accuracy, completeness, and timeliness.
- Legal and Compliance Considerations: Verifying that the data sharing complies with relevant regulations (e.g., GDPR, HIPAA, or industry-specific standards), ensuring privacy and confidentiality agreements are respected.
c) Engage and Communicate with External Stakeholders
Effective communication is key to obtaining data from external sources. This step involves establishing clear communication with external stakeholders, formalizing agreements, and ensuring that the process is efficient:
- Formal Requests for Data: Drafting formal data requests or proposals that specify the types of data required, the intended use, and the timeline for delivery. This may involve:
- Email requests, meetings, or calls to clarify data requirements.
- Formalizing agreements or contracts if the data is proprietary or commercially available.
- Negotiating Terms: Establishing terms of collaboration, including costs (if applicable), data usage rights, timelines, and data privacy/security requirements.
- Data Sharing Agreements: Ensuring that any data shared is done in accordance with legal agreements (e.g., Data Sharing Agreements, Non-Disclosure Agreements). This protects both parties and ensures compliance with data privacy laws.
d) Coordinate Data Collection and Delivery
Once agreements and terms are established, the data collection and delivery process must be carefully coordinated:
- Setting Deadlines: Ensuring that there are clear deadlines for when the external data should be delivered to SayPro, and tracking the delivery schedule.
- Monitoring the Data Flow: Actively monitoring the data delivery to ensure that it aligns with expectations, both in terms of content and timing. If the data is being delivered incrementally, SayPro teams must ensure that they receive all required pieces.
- Technical Assistance: Providing support to external stakeholders if needed, especially if data needs to be formatted or processed in a specific way for integration with SayPro’s systems. This could involve data formatting tools, templates, or specifications.
e) Integration and Validation of External Data
Once the external data is delivered, it needs to be integrated with SayPro’s internal data systems and validated for consistency, quality, and relevance:
- Data Integration: Importing the data into SayPro’s systems (e.g., CRM, database, analytics tools) so that it can be processed and analyzed. This might require the use of APIs, data pipelines, or manual data uploads, depending on the format.
- Data Validation: Ensuring that the external data is accurate and aligns with SayPro’s internal data, checking for discrepancies or errors. If discrepancies are found, it may be necessary to reach back out to the external stakeholder for clarification or correction.
- Testing and Troubleshooting: Testing the data integration to ensure that external data can be used seamlessly in reports, analytics, or dashboards. Troubleshooting may be necessary if data formats are incompatible or if any issues arise during the integration process.
f) Maintaining Ongoing Relationships and Communication
Once external data has been successfully obtained and integrated, it is important to maintain good relationships with the external stakeholders:
- Regular Updates: Keeping external stakeholders informed about how their data is being used and any results or insights generated. This can help strengthen future collaborations.
- Feedback Loop: Providing feedback to external sources if there are issues with the data or if improvements can be made to future data exchanges. This helps maintain the quality and relevance of the data.
- Renewing Agreements: If the data is on a subscription basis or needs to be accessed periodically, coordinating future data requests or renewals is essential to ensure a continuous flow of necessary information.
3. Challenges in External Collaboration and Mitigation Strategies
While collaborating with external stakeholders is necessary, there can be several challenges, including:
- Data Accessibility: Some external data might be proprietary, expensive, or restricted, making it difficult to access. Mitigation might include exploring alternative data sources or negotiating better access terms.
- Data Privacy and Compliance: External data might not align with SayPro’s privacy or regulatory standards, especially if it involves personal or sensitive data. It’s essential to ensure compliance through legal agreements, encryption, and secure data handling practices.
- Quality and Reliability of Data: Not all external data is guaranteed to be accurate or timely. SayPro can mitigate this risk by establishing clear data quality standards upfront, regularly validating external data, and maintaining strong communication with data providers.
- Timeliness: External data providers may not always deliver data on time, which can affect project timelines. SayPro can mitigate this risk by negotiating clear timelines, setting up reminders, and having contingency plans in place.
Conclusion
Effective collaboration with external stakeholders is essential for SayPro to access valuable data that extends beyond its internal systems. Through careful planning, transparent communication, and strong coordination, SayPro can ensure that it gathers high-quality, relevant data that enhances its decision-making, analytics, and reporting capabilities. By following the outlined steps, SayPro not only fills data gaps but also strengthens relationships with key external partners, improving the overall value of the data retrieved and ensuring compliance and integrity in the data management process.
SayPro Collaboration with SayPro Departments: Working closely with different teams within SayPro to ensure all required data is collected in a timely manner.
SayPro Collaboration with SayPro Departments
In our efforts to drive success and streamline operations, SayPro collaborates extensively with various internal departments to ensure that all required data is collected efficiently and on time. This collaboration is key to maintaining accuracy, alignment, and consistency across all projects. By working closely with different teams within SayPro, we ensure that all departments are equipped with the necessary information and resources to meet deadlines and achieve their goals. This process involves clear communication, coordinated efforts, and continuous feedback to enhance productivity and ensure data integrity. Through these strong partnerships, we are able to deliver optimal results for all stakeholders.
SayPro Documentation and Reporting: Preparing reports summarizing the findings from the data retrieval and outlining any areas for further investigation or action.
SayPro Documentation and Reporting: Preparing Reports Summarizing Findings from Data Retrieval and Outlining Areas for Further Investigation or Action
In any data-driven organization, the final step in the data retrieval process is the preparation of comprehensive reports that summarize the findings, assess the success of the data retrieval efforts, and highlight areas that may require further investigation or action. SayPro Documentation and Reporting focuses on providing stakeholders with clear, actionable insights based on the data retrieval process. These reports not only offer a snapshot of how well the data retrieval system is functioning but also provide critical insights into data quality, accuracy, and completeness, as well as highlight areas for improvement.
The reports serve as a communication tool to ensure transparency, keep stakeholders informed, and guide decision-making, particularly when it comes to making improvements, addressing data issues, or taking corrective actions.
1. Purpose of the Report
The main objectives of the documentation and reporting phase are:
- Summarizing Key Findings: To provide a summary of the retrieved data, highlighting key trends, anomalies, and insights that were uncovered during the data retrieval process.
- Assessing Data Quality: To evaluate whether the data retrieved is of sufficient quality—accurate, complete, timely, and consistent—according to pre-defined metrics or quality standards.
- Identifying Issues and Gaps: To identify any data gaps, inaccuracies, or issues that arose during the data retrieval process, and document these for review.
- Recommending Actions: To propose recommendations for further investigation, corrective action, or process improvements.
- Guiding Decision-Making: To provide actionable insights that will guide business decisions, program evaluations, or strategic initiatives.
2. Structure of the Report
The report should be structured in a clear and logical way so that it is easy to understand by both technical and non-technical stakeholders. Below is an outline of the key sections that should be included in a SayPro Data Retrieval Report:
a) Executive Summary
The Executive Summary is a high-level overview of the key findings, issues encountered, and recommendations for further action. This section is intended for stakeholders who may not have the time or need to delve into the detailed report. It should include:
- Overview of the Data Retrieval Process: A brief recap of the data retrieval process, including the sources, methods, and tools used.
- Summary of Key Findings: Key insights uncovered from the retrieved data (e.g., trends, anomalies, critical metrics).
- Main Issues Identified: High-level mention of any issues or gaps encountered in the data retrieval process.
- Recommended Actions: A summary of the recommended next steps based on the findings.
b) Data Retrieval Methodology and Scope
This section describes the methodology and scope of the data retrieval efforts. It provides context for the reader and helps them understand how data was collected, processed, and analyzed. It includes:
- Data Sources: A comprehensive list of the data sources from which information was retrieved, both internal (e.g., CRM systems, databases) and external (e.g., third-party providers, APIs).
- Methodology: A description of the processes, tools, and technologies used to retrieve the data. This includes automated extraction methods (e.g., APIs), manual processes (e.g., data entry), and the ETL (Extract, Transform, Load) processes.
- Timeframe: The time period over which data was retrieved (e.g., daily, monthly, quarterly) and the frequency of data extraction.
- Data Coverage: An outline of the breadth and depth of the data retrieved. Was the data complete for all relevant segments, or were there missing pieces?
c) Key Findings and Analysis
The Key Findings and Analysis section is the core of the report and should present the results of the data retrieval process. This section will be the most detailed and will include:
- Summary of Key Metrics: A breakdown of the key metrics or KPIs (Key Performance Indicators) retrieved. For example, sales performance data, customer engagement metrics, or inventory levels.
- Trends Identified: Any patterns, correlations, or trends uncovered during the analysis of the data. This could include seasonality effects, growth trends, or changes in customer behavior.
- Anomalies or Outliers: Identification of any outliers, anomalies, or unexpected results that may need further investigation. For example, a sudden drop in sales for a specific product category or unexpected spikes in customer complaints.
- Data Quality Assessment: An analysis of the quality of the data retrieved, based on pre-defined quality metrics (e.g., completeness, accuracy, consistency, timeliness). This could include:
- Percentage of missing or incomplete data.
- Instances where the data did not meet the expected accuracy levels.
- Discrepancies between internal data and third-party sources.
- Data Integrity Issues: An overview of any data integrity issues discovered during retrieval, including conflicts between data sources, formatting issues, or inconsistent data points.
d) Issues and Gaps Encountered
This section should provide a detailed account of any issues, errors, or gaps encountered during the data retrieval process. Key areas to address include:
- Technical Issues: Problems with tools, systems, or integration points (e.g., failed API calls, database connection issues, ETL process failures).
- Data Quality Issues: Issues with the accuracy, completeness, or timeliness of the data (e.g., missing customer data, inaccurate transaction records, delayed reporting).
- Access and Permissions: Instances where data could not be retrieved due to access or permission issues (e.g., authorization failures for third-party data).
- Performance Issues: Problems related to slow data retrieval or delays in processing, affecting the timeliness or efficiency of the data.
- Compliance and Security Concerns: If any issues were identified with data privacy, security breaches, or non-compliance with regulatory requirements (e.g., GDPR, HIPAA), these should be highlighted.
e) Root Cause Analysis
For each issue or gap identified, it’s important to conduct a Root Cause Analysis to determine the underlying reasons why the issue occurred. This helps in understanding how to address the issue and prevent it from recurring. The analysis should include:
- Problem Identification: A clear description of the issue or gap.
- Root Cause: The underlying cause of the issue. For example, if data is missing, the root cause might be an issue with the data extraction process, or an error in the source system.
- Impact Analysis: An evaluation of the potential or actual impact of the issue on business operations, decision-making, or reporting.
f) Recommendations for Action
Based on the findings and the issues encountered, the report should include recommendations for further action. This section provides clear guidance on what steps should be taken to address issues, optimize the data retrieval process, and ensure data quality moving forward. Recommendations could include:
- Further Investigation: Areas of the data that require deeper analysis or validation. For example, if discrepancies are found in customer data, a deeper investigation might be required to cross-check data across multiple systems.
- Process Improvements: Suggested improvements in the data retrieval processes. For example, automating certain data checks, improving data validation, or upgrading data extraction tools.
- Technical Enhancements: Any technical improvements needed, such as optimizing the database queries, improving system integrations, or resolving API connectivity issues.
- Data Quality Assurance: Recommendations for improving data quality, such as introducing more stringent validation rules, implementing data cleansing protocols, or improving data collection methods.
- Training and Capacity Building: If issues arose due to user error or lack of knowledge, the report might recommend further training for the team involved in the data retrieval process.
g) Conclusion
The Conclusion should summarize the key takeaways from the report, reinforce the importance of the findings, and restate the primary recommendations for action. It should emphasize the importance of improving the data retrieval process to ensure high-quality, timely, and accurate data for future decision-making.
3. Report Delivery and Stakeholder Communication
Once the report is prepared, it needs to be delivered to the relevant stakeholders. This could include:
- Data Analysts: To inform them of issues and quality concerns and provide them with actionable insights for further analysis.
- Project Managers: To assist in making decisions about ongoing or future projects that depend on accurate data.
- IT/Technical Teams: To help them address any technical issues identified, such as API failures, system integration issues, or performance bottlenecks.
- Executive Leadership: To help them understand the overall success of the data retrieval process, its impact on business operations, and areas that need attention.
The report can be delivered via email, shared in a collaborative platform, or presented in a meeting, depending on the preferences of the organization. Regularly sharing and discussing the reports ensures ongoing communication and collaboration across teams.
Conclusion
SayPro Documentation and Reporting plays a crucial role in ensuring that the data retrieval process is transparent, effective, and continuously improving. By preparing detailed reports that summarize the findings from data retrieval efforts and outline areas for further investigation or action, SayPro ensures that stakeholders are kept informed, issues are addressed, and the organization can move forward with reliable, actionable insights. This process not only improves the quality of decision-making but also builds a culture of accountability and continuous improvement within the data management ecosystem.
SayPro Documentation and Reporting: Creating comprehensive documentation on the data retrieval process, including methodologies used and any issues encountered.
SayPro Documentation and Reporting: Creating Comprehensive Documentation on the Data Retrieval Process, Including Methodologies Used and Any Issues Encountered
Documentation and reporting are fundamental components of the data retrieval process at SayPro. Comprehensive documentation ensures transparency, provides a record of how data is collected and handled, and serves as a valuable resource for troubleshooting, audits, and future improvements. Reporting on the data retrieval process not only captures the methodologies and tools used but also provides a detailed account of any challenges or issues that arise. This process is crucial for maintaining data integrity, improving workflows, and ensuring that all stakeholders have access to reliable information about how data is managed.
Below is a detailed breakdown of how SayPro might approach Documentation and Reporting for data retrieval, covering everything from the methodologies employed to issues faced during the process.
1. Purpose of Documentation and Reporting
Comprehensive documentation serves several purposes:
- Transparency: Ensures that all stakeholders understand how data is retrieved, processed, and stored.
- Audit Trail: Provides a detailed record that can be used for internal and external audits, ensuring compliance with regulatory standards.
- Future Reference: Acts as a reference for troubleshooting issues and optimizing the data retrieval process.
- Continuous Improvement: Helps identify bottlenecks, inefficiencies, and areas for improvement, allowing for optimization in future data retrieval tasks.
2. Structure of Documentation and Reporting
Effective documentation should be clear, structured, and accessible to both technical and non-technical stakeholders. The following sections outline the key components that SayPro should include in its data retrieval documentation.
a) Overview of the Data Retrieval Process
The documentation should begin with an executive summary or an overview that explains the purpose of the data retrieval process and its role within the broader data ecosystem at SayPro. This section could include:
- Objectives: A high-level description of why data retrieval is necessary (e.g., for analytics, reporting, decision-making, or monitoring and evaluation).
- Data Sources: A list of all internal systems (CRM, ERP, data warehouses) and external data sources (third-party providers, APIs) from which data is retrieved.
- Frequency: Details about how often data is retrieved (e.g., real-time, daily, weekly).
b) Methodology and Tools Used
The heart of the documentation will explain the methods and tools used for data retrieval. This section should outline:
- Data Collection Techniques:
- Automated Extraction: Describes the use of APIs, web scraping, or scheduled tasks (ETL processes) to automate data extraction.
- Manual Data Entry: If applicable, it should specify any manual processes used to collect data (e.g., data entry forms, surveys).
- External APIs: Detailing how SayPro integrates with third-party data providers (e.g., payment processors, social media platforms) to pull in external data.
- Data Transformation:
- ETL Process: Describes the Extract, Transform, Load (ETL) process, specifying how raw data is transformed into a usable format before storage.
- Data Cleaning: Details on how the retrieved data is cleaned to ensure it is accurate and complete, including the application of any data validation or normalization procedures.
- Data Storage:
- Data Repositories: Specifies where the retrieved data is stored (e.g., data warehouses, databases, cloud storage).
- Data Formats: Explains the formats in which data is stored (e.g., CSV, JSON, SQL databases).
- Data Security: Provides an overview of security measures to ensure that data is securely retrieved and stored, including encryption and access controls.
c) Data Retrieval Workflows
This section should provide detailed descriptions of the workflows involved in retrieving data. It includes:
- Step-by-Step Data Retrieval Process: A chronological list of steps taken to retrieve, process, and store the data.
- Step 1: Identifying the data requirements (e.g., which metrics or data points are needed).
- Step 2: Connecting to data sources (e.g., querying a database, accessing an API).
- Step 3: Extracting and transforming the data.
- Step 4: Storing the data in appropriate repositories (e.g., data warehouses, cloud storage).
- Flowcharts/Diagrams: Visual representations of the data retrieval process can be included to illustrate the workflow clearly. These can show how data flows from the source to the destination and any transformations or processes in between.
3. Reporting on Issues Encountered
In addition to documenting the methodologies, it is crucial to report any issues encountered during the data retrieval process. This ensures that problems are recorded and addressed in future iterations of the process. This section should include:
a) Types of Issues
The issues encountered during data retrieval can be categorized into several types:
- Data Quality Issues:
- Missing or Incomplete Data: Instances where data was not retrieved fully or was missing essential elements (e.g., missing customer contact information).
- Inaccurate Data: Describes any errors in the data, such as incorrect figures, mismatched records, or data formatting issues.
- System Integration Issues:
- API Failures: If any external APIs failed to provide the requested data or if the connection to a third-party provider was unstable.
- Database Connectivity Problems: Issues related to connecting to internal databases or external data sources.
- ETL Failures: Describes any issues related to the transformation process, such as data not being correctly transformed or loaded into the repository.
- Performance Issues:
- Slow Data Retrieval: Problems with slow extraction or loading of data, which could be related to large data volumes or inefficient query design.
- High Latency: Delays in real-time data retrieval or issues with syncing time-sensitive data.
- Security and Access Control Issues:
- Unauthorized Access: Instances where data access was attempted by unauthorized users, potentially violating security protocols.
- Encryption Failures: Issues related to encrypting sensitive data, such as failure to encrypt data during transmission or at rest.
b) Root Cause Analysis
For each reported issue, the documentation should include:
- Root Cause Analysis: A detailed analysis of why the issue occurred. For example:
- If data was missing, the cause might be traced to an incomplete extraction process or an API timeout.
- If performance was slow, the cause might be traced to inefficient queries or server limitations.
- Impact Assessment: The documentation should describe the potential impact of each issue. For example:
- Data inconsistency could lead to incorrect business decisions.
- API failure could result in missing customer activity data, affecting reporting accuracy.
c) Resolution and Mitigation
For each issue encountered, the documentation should also provide:
- Immediate Actions Taken: Describes how the issue was initially resolved, whether through manual intervention, system fixes, or by rerunning processes.
- Long-Term Solutions: Outlines any systemic improvements made to prevent similar issues in the future, such as automating error handling, optimizing data extraction processes, or upgrading systems.
d) Lessons Learned
After resolving issues, it’s important to document lessons learned:
- Identifying Recurring Issues: Reporting on recurring issues helps in recognizing patterns and implementing permanent fixes.
- Process Improvement: Based on the issues encountered, the team may identify steps to improve the data retrieval process. This could include improving monitoring and alerting systems, enhancing system integrations, or refining ETL workflows.
4. Compliance and Regulatory Reporting
If SayPro is subject to regulatory requirements (e.g., GDPR, HIPAA), it’s important to include compliance checks and reporting:
- Regulatory Compliance: Documentation should indicate how the data retrieval process complies with relevant laws and regulations. This could include details about data retention policies, encryption standards, and the handling of personal data.
- Audit Trails: Ensure that the retrieval process has built-in audit trails that capture who accessed what data and when, ensuring that the system is auditable for regulatory purposes.
5. Version Control and Update Tracking
Documentation is a living document that will evolve over time. A version control system should be used to keep track of changes and updates to the data retrieval process. This section should include:
- Version History: A log of all major changes to the data retrieval processes or methodologies, including who made the changes and the reason for them.
- Change Logs: A detailed record of any updates, fixes, or improvements made to the retrieval process, including any associated issues that were resolved.
6. Reporting on Data Retrieval Performance
To ensure continuous improvement, it’s important to monitor and report on the performance of the data retrieval system:
- Data Retrieval Metrics: These metrics might include the time taken to retrieve and process data, the frequency of data retrieval, and any downtime.
- Performance Benchmarks: Comparing the current performance against historical benchmarks can help identify areas that need optimization.
- User Feedback: Gathering feedback from teams using the data can help ensure the retrieval process meets their needs and expectations.
Conclusion
Comprehensive documentation and reporting on the data retrieval process are crucial for ensuring transparency, accountability, and continuous improvement at SayPro. By thoroughly documenting the methodologies, tools, issues encountered, and resolutions, SayPro can improve the quality and efficiency of its data processes, streamline troubleshooting, and ensure that data is accurately retrieved and aligned with the organization’s objectives. This documentation serves as a vital resource for current operations and as a foundation for future optimizations and audits.
SayPro Data Quality Checks Identifying and rectifying any inconsistencies or gaps in the data.
SayPro Data Quality Checks: Identifying and Rectifying Inconsistencies or Gaps in the Data
Data quality is a critical aspect of data management, as high-quality, reliable data is essential for making informed decisions, performing accurate analyses, and generating trustworthy reports. In the SayPro system, data quality checks are designed to ensure that all data—whether extracted from performance reports, project logs, or evaluation forms—is accurate, consistent, and complete. The process involves identifying and rectifying inconsistencies, gaps, and other quality issues that might compromise the utility of the data.
The following sections provide a detailed breakdown of how SayPro conducts data quality checks, including identifying potential issues, strategies for rectifying them, and ensuring that the data maintains its integrity over time.
1. Types of Data Quality Issues
Before diving into the methods and tools for identifying and rectifying data quality issues, it’s important to first define the common types of data quality problems that may arise in the SayPro system:
a. Inconsistencies
- Definition: Inconsistencies occur when data entries contradict one another, are not aligned with the expected format, or are entered in ways that don’t match predefined standards.
- Examples:
- A task status listed as “In Progress” in one part of the system, but marked as “Completed” in another.
- Date formats that differ (e.g., “MM/DD/YYYY” vs. “YYYY-MM-DD”).
- Different terminology used for the same concept (e.g., “Task Assigned” vs. “Assigned Task”).
b. Missing Data (Data Gaps)
- Definition: Gaps in data occur when required information is absent or incomplete, leaving records lacking key attributes.
- Examples:
- Missing task completion dates or unassigned tasks.
- Incomplete evaluation forms with missing ratings or feedback sections.
- Project logs that lack timestamps or personnel details.
c. Duplicate Data
- Definition: Duplicate data arises when the same record or data point is unintentionally entered multiple times, leading to redundancy.
- Examples:
- Multiple records for the same task or project, often with slightly different details.
- Duplicate entries in performance reports, leading to inaccurate performance tracking.
d. Out-of-Range or Invalid Data
- Definition: Data points that fall outside acceptable ranges or contain values that are not valid according to predefined criteria.
- Examples:
- A task that is recorded as having a negative time spent (e.g., -2 hours).
- Project budgets that exceed the maximum permissible value, based on project constraints.
e. Data Integrity Violations
- Definition: Integrity violations happen when the relationships between data entities are broken or mismatched.
- Examples:
- A task being assigned to a non-existent employee ID.
- A project ID in a task log that does not exist in the project table.
- Evaluation feedback linked to a project that has already been closed or archived.
2. Data Quality Check Processes
To ensure that the data stored within the SayPro system meets the highest standards of quality, the platform employs a set of systematic data quality checks. These checks involve a combination of automated processes and manual review to identify and rectify data issues.
a. Automated Validation Rules
The first layer of defense against data quality issues is automated validation. These validation rules are applied during the data entry or extraction phase to ensure that the data being entered conforms to established standards. They can be implemented within the data input interface or the extraction process and are designed to flag errors as soon as they occur.
- Consistency Validation: Ensures data entries follow standardized formats and naming conventions.
- Example: If a project status is being entered, a validation rule might ensure that the status matches one of the predefined values such as “In Progress,” “Completed,” or “Pending.”
- Completeness Checks: Ensures that all mandatory fields are populated before data is saved or processed.
- Example: A performance report cannot be submitted unless task completion dates and efficiency ratings are entered.
- Range Checks: Ensures that numerical data falls within acceptable boundaries.
- Example: A validation rule checks that hours worked on a task cannot be negative or exceed 24 hours in a day.
- Cross-field Validation: Ensures that interrelated fields are consistent.
- Example: If a task is marked as “Completed,” the completion date must not be empty, and the status field must not allow a value such as “In Progress.”
b. Duplicate Detection
Duplicate data is one of the most common quality issues. SayPro implements automated duplicate detection mechanisms that are triggered during both the data entry and reporting processes.
- Exact Match Check: During data input, a duplicate detection algorithm checks for exact matches of data entries. For example, it checks whether the same project ID and task ID already exist in the system before allowing new entries.
- Fuzzy Matching: When exact matches are not found, fuzzy matching algorithms are employed to identify near-duplicates. This is particularly useful when slight variations (e.g., spelling errors or extra spaces) could result in duplicated records.
- Merge Suggestions: When a duplicate is detected, the system might prompt users to review and merge duplicate records. For example, if two evaluation records for the same employee are identified as duplicates, the user can combine the feedback into a single, comprehensive evaluation record.
c. Data Consistency Checks
Consistency checks verify that the data across different systems or modules within SayPro align with one another. This includes ensuring that interdependent datasets are in sync.
- Cross-Table Validation: For example, if a task is assigned to an employee, a consistency check ensures that the employee exists in the employee database.
- Cross-System Validation: If SayPro integrates with external systems (e.g., project management tools or third-party evaluation platforms), data from those systems is checked against SayPro’s internal datasets for consistency.
- Audit Trail Reviews: SayPro maintains logs of data changes and access, which can be reviewed to ensure that updates to data are consistent with the original context (e.g., project status, task deadlines).
d. Data Gap Identification
Data gaps, or missing information, are identified by comparing current datasets against predefined templates or schemas that outline required fields. Automated tools within SayPro flag records with missing critical information.
- Mandatory Field Checks: During data entry, any required fields that are left empty are flagged, preventing incomplete data from being stored in the system.
- Outlier Detection: Automated systems identify missing or anomalous data by detecting outliers. For example, if a task has no associated “assigned employee,” it is flagged for follow-up.
- Surveys or Feedback Loops: If a report or evaluation form is incomplete (e.g., missing feedback), SayPro can generate automated notifications requesting the missing information from the relevant stakeholders.
e. Manual Review and Exception Handling
While automated processes can catch a large portion of data quality issues, some situations may require human intervention. SayPro facilitates manual review and exception handling through the following steps:
- Quality Assurance (QA) Teams: Dedicated data quality teams can perform spot checks on datasets to ensure that inconsistencies or gaps are identified. They may perform random sampling to ensure that the data integrity is maintained across large datasets.
- Flagging and Notifications: In cases of significant or complex inconsistencies, the system can flag records for manual review. Users or data managers can then investigate, correct, and approve the records as needed.
- User Feedback Loops: End users (e.g., project managers, evaluators, or data analysts) are often involved in identifying missing or incorrect data. SayPro allows users to report inconsistencies or missing data via a feedback system, enabling the platform to generate tickets for review and resolution.
3. Data Quality Rectification
Once data quality issues are identified, the next step is rectification. The goal is to correct inconsistencies, fill gaps, and ensure that the data is brought up to the desired quality standards. This process typically includes:
a. Automated Rectification
In some cases, automated processes can correct data errors. For instance:
- Filling in Missing Values: If certain data points are missing (e.g., task completion dates), the system might automatically populate them based on related or historical data (e.g., by inferring completion dates based on project timelines).
- Data Standardization: The system may automatically standardize data (e.g., converting all date formats to “YYYY-MM-DD”) to ensure consistency.
b. Manual Rectification
For more complex issues, human intervention is often required. This can include:
- Data Review and Update: Users or data managers manually review records flagged by the system for inconsistencies or gaps and make necessary corrections. For example, if an evaluator misses an entry in an evaluation form, the review team might reach out to the evaluator for the missing data.
- Data Merging: In cases of duplicate records, data managers manually merge data from multiple entries to create a single, accurate record.
4. Continuous Monitoring and Feedback
Data quality checks should be an ongoing process. SayPro continuously monitors data quality and provides feedback mechanisms to ensure that the system remains free from quality issues:
- Regular Audits: Routine audits help identify any emerging data quality issues over time and ensure that data standards are maintained.
- Real-time Alerts: Automated alerts notify relevant stakeholders about issues (e.g., missing data, inconsistencies), ensuring timely action.
- User Training: SayPro provides ongoing training and support for users to help them understand data quality best practices and improve data entry processes.
Conclusion
SayPro’s data quality checks are a crucial aspect of maintaining reliable and accurate data throughout its lifecycle. By implementing automated validation rules, detecting duplicates, conducting consistency checks, identifying and filling gaps, and offering manual review processes, SayPro ensures that the data stored in the system is of the highest possible quality. Continuous monitoring, user feedback, and exception handling ensure that any issues are quickly identified and rectified, enabling SayPro to provide trustworthy and actionable insights for project management, performance evaluation, and decision-making.
Saypro Data Quality Checks: Conducting quality assurance procedures to ensure the data is accurate, up-to-date, and aligned with SayPro’s monitoring and evaluation requirements.
SayPro Data Quality Checks: Conducting Quality Assurance Procedures to Ensure Data Accuracy, Timeliness, and Alignment with Monitoring and Evaluation Requirements
Data quality is an essential component of decision-making at SayPro. Without accurate, timely, and relevant data, the effectiveness of analytics, reporting, and strategic decisions can be compromised. To ensure that the retrieved data is of high quality, SayPro needs to implement rigorous Data Quality Checks throughout the entire data lifecycle—from collection and extraction to transformation, storage, and analysis.
Data Quality Checks at SayPro are aimed at ensuring that data is accurate, complete, up-to-date, and aligned with the company’s monitoring and evaluation (M&E) requirements. These quality assurance procedures ensure that the organization can trust the data it uses to make informed decisions, track performance, and evaluate program outcomes.
Below is a detailed breakdown of how SayPro would approach Data Quality Checks.
1. Data Accuracy
Ensuring that data is accurate means verifying that the data reflects the true values it is supposed to represent. Inaccurate data can lead to faulty conclusions, poor business decisions, and missed opportunities.
Techniques for Ensuring Data Accuracy:
- Validation Rules: During data entry, extraction, or transformation, validation rules can be applied to check for errors or inconsistencies. For example, ensuring that numeric fields (e.g., revenue, quantity) do not contain text or that email addresses conform to a standard format (e.g.,
example@domain.com
). - Cross-Checking Data: For important metrics, data can be cross-checked against other trusted sources. For example, comparing sales figures with bank statements or reconciling customer records with other CRM or third-party data sources.
- Data Entry Validation: At the point of data entry, automated checks (e.g., input forms) can ensure that the data entered is correct. This can include required fields (e.g., customer name, address), format checks (e.g., phone numbers), or range checks (e.g., order quantities within reasonable limits).
- Data Consistency across Sources: For data coming from multiple systems (e.g., CRM, ERP, third-party sources), SayPro ensures that the same data across these systems is consistent. Discrepancies can be identified and resolved through comparison and reconciliation procedures.
- Automated Data Audits: Periodic automated audits can identify discrepancies in data values (e.g., if a customer’s order quantity is unusually high) and flag these for review.
2. Data Completeness
Data completeness ensures that all required data is present, and that no essential information is missing. Missing data or incomplete records can skew results and limit analysis.
Techniques for Ensuring Data Completeness:
- Missing Data Detection: Automated systems can be set up to identify missing data fields. For example, a missing “email address” in a customer record, or a “sales amount” in a transaction, can be flagged as incomplete and require follow-up or correction.
- Data Entry Standards: Ensuring that every record contains all necessary fields can be enforced through data entry standards or validation rules. For example, in an order processing system, data related to customer contact details, product ID, order date, and payment status should always be required.
- Historical Data Completion: For retrospective analyses or reports, SayPro might need to fill in missing historical data. Historical data collection strategies and data interpolation techniques can be used to estimate missing values if they are critical for analysis.
- Data Imputation: In cases where some fields are missing but not critical for analysis, imputation techniques (e.g., replacing missing values with mean, median, or predicted values) can be used to maintain completeness without disrupting the analysis.
- Regular Audits: Routine data completeness checks will be scheduled. This includes periodic assessments of databases to identify and fill missing data or records in areas that are crucial for business operations or reporting.
3. Data Timeliness
Data timeliness ensures that data is up-to-date and is available at the right time to make informed decisions. Outdated data can result in missed opportunities, incorrect decisions, or an inability to react quickly to changing conditions.
Techniques for Ensuring Data Timeliness:
- Automated Data Updates: SayPro can implement automatic systems to ensure that data is updated in real-time or at regular intervals (e.g., hourly, daily). For example, sales or inventory data can be updated in real time from a point-of-sale (POS) system or from a live feed in an ERP system.
- Scheduled Data Synchronization: If data is retrieved from multiple sources, ensuring regular synchronization (e.g., once a day, once a week) of the data is crucial to keeping it current. This is important for integrating internal data with external third-party data that may have daily or weekly updates.
- Time-Based Data Validations: Timeliness checks can be set for key records. For instance, for customer orders, SayPro can set validations to ensure that the delivery dates are within a reasonable window of time and that order statuses are regularly updated.
- Timestamping: Every record retrieved should have a timestamp indicating when it was last updated or modified. This helps track the timeliness of the data, ensuring that older or outdated records are flagged for review.
- Real-Time Monitoring: SayPro may implement real-time dashboards or monitoring tools to track the timeliness of data feeds. This allows for early detection of issues where data feeds may have failed or if new data has not been ingested within the expected timeframe.
4. Data Consistency
Data consistency ensures that the data does not conflict with other data points across different systems or repositories. Inconsistent data can cause confusion, misinterpretation, and errors in analysis.
Techniques for Ensuring Data Consistency:
- Standardization of Formats: SayPro can establish standardized data formats for commonly used fields. For example, all date fields should be in the same format (e.g., YYYY-MM-DD), currency should be represented in the same unit (e.g., USD), and addresses should follow a consistent pattern (e.g., street, city, zip code).
- Data Synchronization: Ensuring that data in different systems (e.g., CRM and ERP) are aligned and synchronized regularly to prevent inconsistencies between systems. If a customer’s address is updated in one system, it should be reflected across all systems.
- Conflict Resolution: Automated conflict resolution processes can be put in place to flag data discrepancies, allowing for reconciliation of conflicting data across different sources. For example, if one system records a customer’s name differently from another, it should be flagged for manual review.
- Data Reconciliation: SayPro can set up processes to compare data from different systems and resolve inconsistencies. For example, comparing inventory data from the ERP system with actual stock levels could identify discrepancies that need to be addressed.
- Data Audits: Regular audits and quality checks of key data elements (e.g., product details, client data) will be performed to ensure consistency between data repositories.
5. Data Relevance
Data relevance ensures that the data being used is aligned with SayPro’s monitoring and evaluation (M&E) requirements and business objectives. Irrelevant or unnecessary data can clutter the analysis and lead to incorrect conclusions.
Techniques for Ensuring Data Relevance:
- Alignment with KPIs: Data should be aligned with Key Performance Indicators (KPIs) and business objectives. For instance, SayPro may focus on customer acquisition, satisfaction, and retention as key performance metrics, so all relevant data should support these metrics.
- Data Mapping and Requirements Analysis: SayPro’s M&E team would perform a thorough analysis of the business goals and the data required to evaluate them. This ensures that only relevant data is collected, eliminating unnecessary or non-actionable data from the system.
- Filtering and Segmentation: SayPro can apply filters to focus on the most relevant data subsets. For example, sales data may only be analyzed for a specific region or period based on business needs, helping to avoid unnecessary data overload.
- Monitoring and Evaluation Frameworks: SayPro’s M&E teams may define clear frameworks and guidelines for identifying and collecting only relevant data that helps track progress on projects, programs, or initiatives.
6. Data Compliance and Governance
Compliance and governance checks ensure that data adheres to internal and external regulations (e.g., GDPR, HIPAA) and follows organizational policies regarding data privacy, security, and usage.
Techniques for Ensuring Data Compliance:
- Data Privacy Checks: SayPro will implement robust data privacy policies, including checks to ensure that sensitive data (e.g., personally identifiable information, financial data) is handled in compliance with regulations like GDPR or CCPA.
- Audit Trails: Keeping detailed records of data access, usage, and modification through audit trails helps ensure that the data is used appropriately and in accordance with company policies and regulations.
- Regulatory Compliance Audits: Regular audits should be performed to ensure that all data handling practices comply with industry-specific regulations and laws.
Conclusion
Effective Data Quality Checks at SayPro are critical for maintaining trust in the organization’s data and ensuring that it is suitable for analysis, reporting, and decision-making. By implementing systematic procedures to verify the accuracy, completeness, timeliness, consistency, relevance, and compliance of data, SayPro ensures that its data meets the highest standards. This not only improves the quality of insights derived from data but also helps align data with monitoring and evaluation requirements, ensuring it supports both strategic and operational goals.
- Validation Rules: During data entry, extraction, or transformation, validation rules can be applied to check for errors or inconsistencies. For example, ensuring that numeric fields (e.g., revenue, quantity) do not contain text or that email addresses conform to a standard format (e.g.,
SayPro Data Organization: Storing data according to the established SayPro Data Management guidelines to maintain consistency and integrity.
SayPro Data Organization: Storing Data According to Established SayPro Data Management Guidelines
Data organization is a critical component of any data management framework, ensuring that data is stored in a structured, consistent, and accessible manner. For SayPro, which handles a wide range of data from sources like performance reports, project logs, and evaluation forms, organizing the data according to established SayPro Data Management guidelines is essential for maintaining data integrity, supporting efficient retrieval, and ensuring the overall quality of the data ecosystem.
The following sections provide a comprehensive breakdown of how SayPro ensures the consistent and organized storage of data:
1. Establishing Data Management Guidelines
The first step in organizing data is to define clear guidelines that standardize how data is handled across the system. SayPro Data Management guidelines address several core principles:
- Consistency: Ensuring that data is formatted and stored in a uniform manner, making it easy to aggregate and compare data from different sources.
- Integrity: Ensuring that data is accurate, complete, and reliable by establishing processes for validation, error-checking, and handling missing or incomplete data.
- Accessibility: Structuring data in such a way that it is easily retrievable for analysis, reporting, and decision-making.
- Security and Compliance: Storing data in compliance with privacy regulations (e.g., GDPR, HIPAA) and ensuring it is protected from unauthorized access.
- Scalability: Preparing the data storage system to handle increased volumes of data over time without compromising performance.
These guidelines set the foundation for how SayPro handles data across its lifecycle.
2. Data Structuring and Categorization
Once data is collected and processed, it needs to be categorized and structured for storage. This involves creating a well-defined schema that reflects the relationships between different types of data and ensures that data is stored logically.
a. Data Entities and Relationships
Data in SayPro can be categorized into various entities (or objects) based on the types of data sources. The core data entities might include:
- Projects: Data related to specific projects, including project names, IDs, deadlines, and overall status.
- Tasks: Information on individual tasks within projects, including task descriptions, assignees, deadlines, and progress status.
- Performance Metrics: Data about the efficiency, productivity, and quality of task completion within a project.
- Evaluations: Feedback, ratings, and qualitative comments collected from stakeholders or users.
- Resources: Data regarding resource allocation, such as personnel, tools, or budget associated with projects and tasks.
b. Data Types and Formats
The data is categorized into different types depending on its nature:
- Structured Data: Data that fits neatly into a predefined model or schema, such as project details (e.g., project ID, task name, task status). This type of data is stored in relational databases or structured file formats (CSV, Excel).
- Unstructured Data: Data such as free-text feedback, logs, and comments from evaluation forms or project reports, typically stored in NoSQL databases or document-based formats (JSON, XML, or plain text files).
- Semi-Structured Data: Data that has some structure but doesn’t fit completely into a rigid schema. This might include emails, PDFs, or web-based forms that combine structured fields (e.g., ratings) and unstructured text (e.g., feedback).
c. Data Taxonomy
SayPro creates a data taxonomy, grouping data into relevant categories (e.g., “Project Data”, “Task Data”, “Performance Data”, “Evaluation Data”). Each category will have predefined attributes that are consistent across the data. For example:
- Project Data: Project ID, Name, Deadline, Budget, Stakeholders
- Task Data: Task ID, Task Name, Assigned Personnel, Start Date, End Date, Status
- Performance Data: Completion Rate, Time Taken, Resource Utilization, Efficiency Metrics
- Evaluation Data: Feedback, Rating, Evaluation Date, Evaluator ID
This structure ensures that data from different sources is stored in an organized way, making it easier to access, analyze, and report.
3. Data Storage Models
SayPro employs different storage models to optimize data storage based on the type and volume of data. The main models include:
a. Relational Databases (SQL-based)
For structured data (e.g., task logs, project details, performance metrics), SayPro uses relational databases such as MySQL, PostgreSQL, or Microsoft SQL Server. In these databases:
- Tables: Each data entity (such as Projects, Tasks, Performance Metrics) is represented by a table, with rows for individual data records and columns for attributes (e.g., task ID, task description, assignee).
- Indexes: Indexing is used to speed up query performance, particularly for frequently accessed fields such as task IDs, project names, and employee IDs.
- Relationships: Foreign keys are used to define relationships between tables, ensuring data integrity. For instance, a task table might have a foreign key linking each task to a specific project.
b. NoSQL Databases
For semi-structured and unstructured data (e.g., feedback comments, project logs), SayPro uses NoSQL databases like MongoDB or Elasticsearch. In these databases:
- Document-based storage: Data is stored in flexible formats (like JSON or BSON), allowing for complex and nested structures. This is ideal for storing unstructured or semi-structured data, such as project logs or evaluation feedback, where the attributes may vary by entry.
- Full-text Search: NoSQL databases often include full-text search capabilities, enabling SayPro to quickly retrieve text-based data such as project notes or feedback comments.
c. Cloud Storage
For large volumes of data, such as project reports, logs, and documents, SayPro may use cloud storage services like AWS S3, Google Cloud Storage, or Azure Blob Storage. This is suitable for storing large files (e.g., PDF reports, images, or backup files) that don’t need to be queried in real-time but require secure and scalable storage.
d. Data Warehouses
For long-term storage and analytics, SayPro uses data warehouses (such as Amazon Redshift or Google BigQuery). Data warehouses store large amounts of historical data and support complex analytical queries across large datasets. This is particularly useful for generating insights and reports over long periods (e.g., project performance trends or resource utilization metrics over months or years).
4. Data Integrity and Validation
Maintaining data integrity is a fundamental aspect of data organization in SayPro. To ensure that the data stored is accurate, consistent, and reliable, SayPro implements the following strategies:
a. Data Validation Rules
- Validation during data entry: Whenever data is entered into the system (whether through manual input or automated extraction), predefined validation rules check for accuracy. For example, task completion dates cannot precede the project start date.
- Referential Integrity: Ensuring that foreign key relationships between data tables are respected. For instance, if a task record refers to a specific project ID, that project must exist in the “Projects” table, otherwise the task record is rejected.
b. Automated Data Quality Checks
- Error Detection: Automated processes run on a scheduled basis to detect anomalies or discrepancies in the data. These processes may check for duplicate entries, missing values, or values outside expected ranges.
- Data Consistency: SayPro ensures that data is consistent across systems. For example, if performance data is stored in both a relational database and a cloud storage system, consistency checks will ensure that both versions of the data are synchronized.
5. Data Security and Access Control
SayPro uses robust security protocols to ensure that data is protected and that access is controlled according to predefined roles and permissions.
- Encryption: All data stored within SayPro is encrypted both at rest and during transit to prevent unauthorized access. Encryption ensures that sensitive information (such as performance metrics or evaluation feedback) remains secure.
- Role-based Access Control (RBAC): Access to data is controlled based on the roles assigned to different users. For instance, project managers might have access to all project data, while evaluators may only have access to evaluation forms and feedback.
- Audit Trails: SayPro maintains an audit trail that logs all access to and modifications of data. This provides transparency and accountability, allowing administrators to track who accessed or changed specific data records.
6. Backup and Disaster Recovery
To ensure data resilience, SayPro implements regular backup procedures and a disaster recovery plan. This involves:
- Automated Backups: Data is backed up regularly (e.g., daily, weekly) to prevent loss of data due to system failures.
- Versioning: Historical versions of key data entities (e.g., performance reports) are stored, allowing for data recovery in case of accidental deletion or corruption.
- Disaster Recovery Protocols: A comprehensive disaster recovery plan is in place to quickly restore data from backups in the event of system failure or data corruption.
Conclusion
SayPro’s data organization strategy relies on a set of well-defined guidelines that ensure data is stored consistently, securely, and in a manner that maintains its integrity. By structuring data according to predefined categories, using suitable storage models, and implementing robust security and validation procedures, SayPro ensures that the data remains reliable and accessible for analysis and decision-making. With scalable storage solutions and automated checks, SayPro can continue to manage large volumes of data efficiently while maintaining high standards of data quality and compliance.
SayPro Data Organization: Organizing the retrieved data into accessible formats and repositories for easy analysis.
SayPro Data Organization: Organizing Retrieved Data into Accessible Formats and Repositories for Easy Analysis
Data organization is a critical aspect of managing large volumes of data in any organization, including SayPro. After data has been retrieved from various internal systems and external sources, it needs to be effectively structured and stored to ensure it can be easily accessed, analyzed, and utilized for decision-making. The process of data organization involves several steps that focus on structuring data in a manner that maximizes its usability and efficiency for various stakeholders within the organization. Below is a detailed breakdown of how SayPro might organize its retrieved data for easy analysis.
1. Data Structuring and Categorization
Once data is retrieved, it must be categorized and structured in ways that align with the organization’s needs and business objectives. This involves determining what type of data is being retrieved and ensuring it is organized appropriately.
Types of Data
- Transactional Data: This includes data related to individual transactions, such as customer orders, financial records, inventory updates, or service tickets. This data typically includes timestamped events and may need to be structured in a time-series format for analysis.
- Reference Data: This includes static or slowly changing data such as customer information, product categories, employee records, and geographic data. This data is typically used to provide context for transactional data.
- Operational Data: Data from internal operations such as supply chain logistics, manufacturing, and sales data that need to be categorized and stored based on different business operations.
Categorization of Data
- By Department: Data can be organized based on which department it pertains to (e.g., HR, Sales, Finance, Operations).
- By Source: Data can be categorized by the system or source from which it was extracted (e.g., CRM, ERP, External API).
- By Time: Time-based data (e.g., sales performance, traffic, or financial transactions) is often organized chronologically, allowing for trends, patterns, and seasonality to be easily observed.
Data Normalization
- Standardizing Data: Data retrieved from different sources may not have the same format or unit of measurement. SayPro must standardize data types, formats (e.g., date formats, currencies), and measurement units to ensure consistency across datasets.
- Data Cleansing: This process is used to remove or correct inaccurate, incomplete, or duplicate data. Ensuring high data quality at this stage is vital for making reliable business decisions.
2. Data Storage and Repository Design
Organizing data into well-structured repositories ensures that it is easy to access, secure, and ready for analysis. The two key aspects of organizing data storage are choosing the right type of storage infrastructure and setting up a logical schema for the data.
Types of Storage Repositories
- Data Warehouses: A data warehouse is an integrated repository designed for analytical purposes. It consolidates data from different internal systems (such as CRM, ERP) and external sources into a single location. Data warehouses use a schema model to structure the data, making it easier for analysts and business users to query and analyze large datasets.
- Data Lakes: For more unstructured or semi-structured data (e.g., logs, multimedia, or sensor data), SayPro may use a data lake. Data lakes allow storage of raw data without requiring prior structuring, offering flexibility for future processing or analysis.
- Databases: Relational databases (e.g., MySQL, PostgreSQL, or Oracle) may be used for operational storage, where quick retrieval of structured data is needed. These databases are designed to support transactional processes and are optimized for high-volume, high-speed operations.
- Cloud Storage: SayPro may opt for cloud-based data storage solutions like Amazon S3, Microsoft Azure, or Google Cloud Storage for scalability and cost efficiency. Cloud-based storage allows for on-demand access to data, making it easy to scale storage as needed.
Data Schemas and Tables
- Star Schema: A common design for data warehouses, where data is organized into fact tables (transactional data) and dimension tables (reference data like customers, time, or products). This design simplifies complex queries and is widely used in business intelligence systems.
- Snowflake Schema: A variant of the star schema, which normalizes the dimension tables into multiple related tables to reduce redundancy. This design can be more efficient in terms of storage but may be more complex to query.
- Flat Tables: For less complex data sets or for quick reports, flat tables might be used, where all data is stored in a single table without relational structures.
3. Data Indexing and Optimization
Efficient data retrieval is crucial for ensuring that users can access the data quickly when performing analyses. Data indexing and optimization techniques are employed to enhance query performance.
Indexing Data
- Primary Indexes: These are used to uniquely identify records within a table, typically through a primary key (e.g., customer ID, order number). Indexes help speed up lookups and join operations.
- Secondary Indexes: These indexes are created on non-primary key columns (e.g., product name, order date) to enable faster searching and filtering based on these attributes.
- Composite Indexes: Sometimes, it is necessary to index multiple columns together (e.g., customer ID and order date). Composite indexes help improve query performance on complex filters or joins.
Query Optimization
- Partitioning Data: Data can be partitioned across multiple databases or storage systems based on attributes such as time, geographic region, or data category. Partitioning improves performance and allows for better scalability.
- Denormalization: While normalization (storing data in smaller tables) reduces redundancy, it may lead to slow query performance. In some cases, denormalizing data (storing data in fewer, larger tables) can improve read speed by reducing the need for joins.
4. Data Access and Security Controls
Ensuring that the right people can access the organized data is vital for both usability and security.
Data Access Layers
- Business Intelligence Tools: Tools like Power BI, Tableau, or Looker are often used for end-users to access organized data in an easy-to-understand format through dashboards and reports. These tools allow for self-service analytics, so departments can query the data without requiring heavy IT intervention.
- Data APIs: SayPro may expose certain datasets via APIs, allowing external applications or internal systems to retrieve data programmatically for further processing or integration.
Role-Based Access Control (RBAC)
- Data Permissions: SayPro should implement robust access controls to ensure that only authorized users can access sensitive data. Role-based access ensures that employees only have access to the data they need to perform their jobs.
- Encryption: Data at rest and in transit should be encrypted to protect against unauthorized access, especially when dealing with sensitive or personal information.
Data Lineage and Auditing
- Tracking Changes: It’s important to track changes to the data, such as who accessed or modified it, and what transformations were applied. Data lineage tools can help visualize this process and ensure that the data is accurate and auditable.
- Audit Trails: Maintaining an audit trail ensures compliance with regulatory requirements and allows for the tracking of how data is used and changed over time.
5. Data Governance and Quality Assurance
Data governance ensures that the retrieved and organized data meets the quality standards required for analysis and decision-making.
Data Stewardship
- Data Steward: A data steward may be responsible for managing the quality and accuracy of the data within specific repositories. This role ensures that data is properly maintained and that data quality issues are addressed in a timely manner.
Quality Assurance Processes
- Data Validation: Data validation rules are used to ensure that the data entered into the system meets certain quality standards (e.g., checking for missing values, data range issues, or type mismatches).
- Consistency Checks: Regular checks can be performed to ensure that the data remains consistent and correct over time, especially when integrating data from multiple sources.
6. Data Visualization and Reporting
After data is organized, SayPro can use various tools to transform raw data into actionable insights. Organizing the data in a way that allows for intuitive visualization is crucial.
Dashboard Creation
- Interactive Dashboards: Tools like Power BI or Tableau enable the creation of interactive dashboards that provide insights into key metrics, such as sales performance, customer satisfaction, or financial health. These dashboards can be customized based on the needs of different departments.
- Automated Reports: Scheduled reports that automatically update and are sent to stakeholders (e.g., weekly sales reports, monthly performance reviews) allow teams to monitor metrics regularly.
Data Analytics and Machine Learning Models
- Data Mining: Organizing data in an accessible format allows SayPro’s data scientists to apply data mining techniques to identify trends, patterns, and correlations in the data.
- Predictive Analytics: By organizing and structuring the data correctly, SayPro can apply machine learning models to predict future outcomes, such as sales forecasts or customer churn.
Conclusion
Data organization is a critical step in ensuring that SayPro can make the most of the data it collects. By structuring, storing, indexing, and securing data in well-defined repositories, SayPro enables its teams to easily access, analyze, and extract valuable insights. A well-organized data ecosystem ensures that the organization can maintain high data quality, facilitate quick decision-making, and optimize business operations. Effective data organization also enables a scalable infrastructure that can grow as SayPro’s data needs evolve.
SayPro Data Organization: Organizing the retrieved data into accessible formats and repositories for easy analysis.
SayPro Data Organization: Organizing Retrieved Data into Accessible Formats and Repositories for Easy Analysis
Data organization is a critical aspect of managing large volumes of data in any organization, including SayPro. After data has been retrieved from various internal systems and external sources, it needs to be effectively structured and stored to ensure it can be easily accessed, analyzed, and utilized for decision-making. The process of data organization involves several steps that focus on structuring data in a manner that maximizes its usability and efficiency for various stakeholders within the organization. Below is a detailed breakdown of how SayPro might organize its retrieved data for easy analysis.
1. Data Structuring and Categorization
Once data is retrieved, it must be categorized and structured in ways that align with the organization’s needs and business objectives. This involves determining what type of data is being retrieved and ensuring it is organized appropriately.
Types of Data
- Transactional Data: This includes data related to individual transactions, such as customer orders, financial records, inventory updates, or service tickets. This data typically includes timestamped events and may need to be structured in a time-series format for analysis.
- Reference Data: This includes static or slowly changing data such as customer information, product categories, employee records, and geographic data. This data is typically used to provide context for transactional data.
- Operational Data: Data from internal operations such as supply chain logistics, manufacturing, and sales data that need to be categorized and stored based on different business operations.
Categorization of Data
- By Department: Data can be organized based on which department it pertains to (e.g., HR, Sales, Finance, Operations).
- By Source: Data can be categorized by the system or source from which it was extracted (e.g., CRM, ERP, External API).
- By Time: Time-based data (e.g., sales performance, traffic, or financial transactions) is often organized chronologically, allowing for trends, patterns, and seasonality to be easily observed.
Data Normalization
- Standardizing Data: Data retrieved from different sources may not have the same format or unit of measurement. SayPro must standardize data types, formats (e.g., date formats, currencies), and measurement units to ensure consistency across datasets.
- Data Cleansing: This process is used to remove or correct inaccurate, incomplete, or duplicate data. Ensuring high data quality at this stage is vital for making reliable business decisions.
2. Data Storage and Repository Design
Organizing data into well-structured repositories ensures that it is easy to access, secure, and ready for analysis. The two key aspects of organizing data storage are choosing the right type of storage infrastructure and setting up a logical schema for the data.
Types of Storage Repositories
- Data Warehouses: A data warehouse is an integrated repository designed for analytical purposes. It consolidates data from different internal systems (such as CRM, ERP) and external sources into a single location. Data warehouses use a schema model to structure the data, making it easier for analysts and business users to query and analyze large datasets.
- Data Lakes: For more unstructured or semi-structured data (e.g., logs, multimedia, or sensor data), SayPro may use a data lake. Data lakes allow storage of raw data without requiring prior structuring, offering flexibility for future processing or analysis.
- Databases: Relational databases (e.g., MySQL, PostgreSQL, or Oracle) may be used for operational storage, where quick retrieval of structured data is needed. These databases are designed to support transactional processes and are optimized for high-volume, high-speed operations.
- Cloud Storage: SayPro may opt for cloud-based data storage solutions like Amazon S3, Microsoft Azure, or Google Cloud Storage for scalability and cost efficiency. Cloud-based storage allows for on-demand access to data, making it easy to scale storage as needed.
Data Schemas and Tables
- Star Schema: A common design for data warehouses, where data is organized into fact tables (transactional data) and dimension tables (reference data like customers, time, or products). This design simplifies complex queries and is widely used in business intelligence systems.
- Snowflake Schema: A variant of the star schema, which normalizes the dimension tables into multiple related tables to reduce redundancy. This design can be more efficient in terms of storage but may be more complex to query.
- Flat Tables: For less complex data sets or for quick reports, flat tables might be used, where all data is stored in a single table without relational structures.
3. Data Indexing and Optimization
Efficient data retrieval is crucial for ensuring that users can access the data quickly when performing analyses. Data indexing and optimization techniques are employed to enhance query performance.
Indexing Data
- Primary Indexes: These are used to uniquely identify records within a table, typically through a primary key (e.g., customer ID, order number). Indexes help speed up lookups and join operations.
- Secondary Indexes: These indexes are created on non-primary key columns (e.g., product name, order date) to enable faster searching and filtering based on these attributes.
- Composite Indexes: Sometimes, it is necessary to index multiple columns together (e.g., customer ID and order date). Composite indexes help improve query performance on complex filters or joins.
Query Optimization
- Partitioning Data: Data can be partitioned across multiple databases or storage systems based on attributes such as time, geographic region, or data category. Partitioning improves performance and allows for better scalability.
- Denormalization: While normalization (storing data in smaller tables) reduces redundancy, it may lead to slow query performance. In some cases, denormalizing data (storing data in fewer, larger tables) can improve read speed by reducing the need for joins.
4. Data Access and Security Controls
Ensuring that the right people can access the organized data is vital for both usability and security.
Data Access Layers
- Business Intelligence Tools: Tools like Power BI, Tableau, or Looker are often used for end-users to access organized data in an easy-to-understand format through dashboards and reports. These tools allow for self-service analytics, so departments can query the data without requiring heavy IT intervention.
- Data APIs: SayPro may expose certain datasets via APIs, allowing external applications or internal systems to retrieve data programmatically for further processing or integration.
Role-Based Access Control (RBAC)
- Data Permissions: SayPro should implement robust access controls to ensure that only authorized users can access sensitive data. Role-based access ensures that employees only have access to the data they need to perform their jobs.
- Encryption: Data at rest and in transit should be encrypted to protect against unauthorized access, especially when dealing with sensitive or personal information.
Data Lineage and Auditing
- Tracking Changes: It’s important to track changes to the data, such as who accessed or modified it, and what transformations were applied. Data lineage tools can help visualize this process and ensure that the data is accurate and auditable.
- Audit Trails: Maintaining an audit trail ensures compliance with regulatory requirements and allows for the tracking of how data is used and changed over time.
5. Data Governance and Quality Assurance
Data governance ensures that the retrieved and organized data meets the quality standards required for analysis and decision-making.
Data Stewardship
- Data Steward: A data steward may be responsible for managing the quality and accuracy of the data within specific repositories. This role ensures that data is properly maintained and that data quality issues are addressed in a timely manner.
Quality Assurance Processes
- Data Validation: Data validation rules are used to ensure that the data entered into the system meets certain quality standards (e.g., checking for missing values, data range issues, or type mismatches).
- Consistency Checks: Regular checks can be performed to ensure that the data remains consistent and correct over time, especially when integrating data from multiple sources.
6. Data Visualization and Reporting
After data is organized, SayPro can use various tools to transform raw data into actionable insights. Organizing the data in a way that allows for intuitive visualization is crucial.
Dashboard Creation
- Interactive Dashboards: Tools like Power BI or Tableau enable the creation of interactive dashboards that provide insights into key metrics, such as sales performance, customer satisfaction, or financial health. These dashboards can be customized based on the needs of different departments.
- Automated Reports: Scheduled reports that automatically update and are sent to stakeholders (e.g., weekly sales reports, monthly performance reviews) allow teams to monitor metrics regularly.
Data Analytics and Machine Learning Models
- Data Mining: Organizing data in an accessible format allows SayPro’s data scientists to apply data mining techniques to identify trends, patterns, and correlations in the data.
- Predictive Analytics: By organizing and structuring the data correctly, SayPro can apply machine learning models to predict future outcomes, such as sales forecasts or customer churn.
Conclusion
Data organization is a critical step in ensuring that SayPro can make the most of the data it collects. By structuring, storing, indexing, and securing data in well-defined repositories, SayPro enables its teams to easily access, analyze, and extract valuable insights. A well-organized data ecosystem ensures that the organization can maintain high data quality, facilitate quick decision-making, and optimize business operations. Effective data organization also enables a scalable infrastructure that can grow as SayPro’s data needs evolve.