Your cart is currently empty!
SayPro Data Collection and Analysis
SayPro is a Global Solutions Provider working with Individuals, Governments, Corporate Businesses, Municipalities, International Institutions. SayPro works across various Industries, Sectors providing wide range of solutions.
Email: info@saypro.online Call/WhatsApp: Use Chat Button 👇
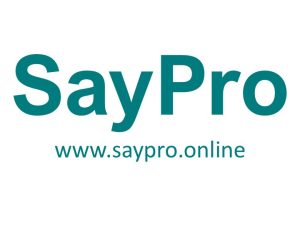
Data Collection
a. Set Up Data Collection Tools
- Choose a Reliable Survey Platform: Use trusted tools like Google Forms, SurveyMonkey, or Typeform to distribute your survey and automatically collect responses. These platforms often have built-in features to help you track submissions and gather data efficiently.
- Customization: Customize the survey to capture responses accurately based on the specific questions you’re asking.
- Data Exporting: Ensure that the tool you use allows easy exporting of data into Excel, CSV, or SPSS formats for analysis.
b. Monitor Survey Participation
- Track Responses in Real Time: Keep an eye on the real-time progress of responses. Some platforms offer live tracking, where you can see how many responses have been submitted and how many are still pending.
- Respondent Segmentation: Categorize respondents based on relevant demographics (e.g., age, region, education level) to ensure you get a diverse sample.
c. Ensure Complete Data Collection
- Use Required Fields: In the survey, mark essential questions as required to prevent missing responses.
- Follow Up on Non-Respondents: Reach out to participants who started the survey but haven’t completed it, using the strategies discussed earlier.
- Allow for Edits: If applicable, enable participants to edit their responses after submission, in case they realize they’ve missed something important.
2. Data Validation
a. Check for Duplicate Responses
- Prevent Multiple Submissions: Some survey platforms allow you to limit submissions per respondent by tracking IP addresses or using unique codes.
- Detect Duplicate Entries: Manually or with the help of software, check for duplicate responses from the same individuals (e.g., same answers or similar timestamps).
b. Evaluate Incomplete or Invalid Responses
- Missing Data: Identify responses that have missing or blank fields (except when they are optional).
- Outliers or Inconsistent Answers: Identify extreme outliers or contradictory answers (e.g., “I prefer online learning” in one question but “I prefer in-person learning” in another). Decide whether to exclude these or follow up for clarification.
- Logic Checks: If your survey uses skip logic (e.g., if you answer “Yes” to question 3, you go to question 4), make sure that the responses follow the correct logic.
c. Correct Errors
- If any errors are found in data (e.g., incorrect responses to open-ended questions or errors in numerical data), correct them where possible or reach out to the respondent for clarification.
3. Data Compilation
a. Export Data
- Export to the Correct Format: Export the survey data into a manageable format (Excel, CSV, or SPSS). This will allow you to manipulate and analyze the data in various ways.
- Organize Responses: Organize your data in a spreadsheet where each row represents a respondent and each column represents a survey question. This will make it easy to identify trends.
b. Clean the Data
- Remove Unnecessary Data: Eliminate any irrelevant or incomplete data, such as responses from non-target groups (e.g., someone who didn’t fit your demographic criteria).
- Standardize Responses: If there are open-ended questions, categorize or code responses into common themes. For example, if people wrote different variations of “online learning,” group those responses together under one category like “Preference for Online Learning.”
4. Data Analysis
a. Quantitative Analysis (Closed-Ended Questions)
- Descriptive Statistics: Calculate measures such as mean, median, mode, and standard deviation to summarize key metrics.
- Frequencies: Identify how often each answer was selected. For example, how many people preferred online learning vs. in-person learning.
- Cross-Tabulation: If needed, analyze relationships between multiple variables (e.g., are younger students more likely to prefer online learning than older students?).
- Bar/ Pie Charts: Create visual representations of the data to make trends and preferences clearer.
b. Qualitative Analysis (Open-Ended Questions)
- Categorize Responses: For open-ended questions, group similar responses into themes (e.g., responses mentioning “flexibility” could be grouped under a theme of “flexibility in learning”).
- Content Analysis: Identify keywords or phrases that are mentioned frequently in the responses to highlight common themes.
- Sentiment Analysis: If needed, you can analyze the tone of open-ended responses (positive, neutral, negative) to gauge overall sentiment on specific topics.
c. Cross-Tabulation and Trend Analysis
- If you have multiple demographic variables (e.g., age, gender, education level), use cross-tabulation to understand how different groups responded to each survey question.
- Example: “How do responses from high school students differ from responses from college students regarding preferred teaching methods?”
5. Data Integrity and Ethics
a. Ensure Anonymity
- Ensure that all collected data maintains the anonymity of participants if promised. Remove any identifying information unless required for analysis and ensure participants are aware of how their data will be used.
b. Adhere to Ethical Standards
- Consent: Ensure that you have consent from respondents to use their data for the intended purpose.
- Data Security: Store all data securely and ensure that it is protected from unauthorized access.
6. Preparing for Reporting
a. Prepare Visuals
- Graphs and Charts: Create visuals (graphs, pie charts, bar charts) that present the findings clearly and effectively for easy interpretation.
- Summarize Key Metrics: Highlight the most important statistics that will be useful for decision-making or for the stakeholders.
b. Identify Key Insights
- Review your analysis and identify the key findings or trends that directly relate to the educational needs, challenges, and preferences of your target groups.
- These insights will guide the next steps in your research and help in shaping educational strategies or policies.
1. Clean & Prepare the Data
Ensure:
- No duplicates or blank entries.
- All variables (questions) are labeled clearly.
- Open-ended responses are grouped into common themes for qualitative analysis.
📊 2. Descriptive Statistics (Basic Overview)
Use tools like Excel, Google Sheets, or SPSS to compute:
- Frequencies & Percentages – How many respondents chose each option?
- Mean (Average) – E.g., average rating of current education satisfaction on a 1–5 scale.
- Mode/Median – Most common response and middle value in satisfaction, preferences, etc.
Example Insight:
62% of students preferred blended learning over purely online or in-person formats.
📈 3. Cross-Tabulation (Comparing Groups)
Helps compare responses across demographics like age, location, or educator vs. student.
Example:
- Cross-tab student age with learning format preference.
- 18–25-year-olds: 70% prefer online learning.
- 40+ age group: 60% prefer in-person classes.
This tells you how different groups experience or prefer education differently.
📉 4. Trend Analysis
If you have data over time or from multiple rounds, identify shifts in opinions.
Example:
Compared to January, April responses showed a 25% increase in demand for career readiness programs among high school students.
📚 5. Thematic Analysis (Open-Ended Responses)
Use word clouds or coding to identify common phrases and ideas.
Steps:
- Read through open responses.
- Group similar ideas into themes (e.g., “lack of resources,” “language barriers,” “tech training needed”).
- Count how many times each theme is mentioned.
Example Themes:
- 45% mentioned “need for career guidance.”
- 38% expressed “difficulty with internet access.”
📌 6. Correlation & Relationship Testing (Advanced)
Use correlation to test if two variables are related:
- In SPSS or Excel, calculate Pearson correlation between variables.
Example:
Positive correlation (r = 0.63) between satisfaction with teacher support and student performance perception.
This means students who feel more supported by teachers also tend to feel they perform better.
💡 7. Key Insights Summary
Based on your analysis, here’s how you could summarize your insights:
🔑 Key Findings:
- Student Preferences: 62% favor blended learning; younger students lean toward online.
- Educator Needs: Teachers need more digital teaching tools and training (noted in 52% of educator responses).
- Broad Trends:
- Career-focused programs are in rising demand.
- There’s a growing concern about digital inequality.
- Emotional well-being and support services were highlighted by both students and teachers.
Leave a Reply