Your cart is currently empty!
SayPro Data Analysis:Analyze quantitative and qualitative data using statistical tools and thematic analysis.
SayPro is a Global Solutions Provider working with Individuals, Governments, Corporate Businesses, Municipalities, International Institutions. SayPro works across various Industries, Sectors providing wide range of solutions.
Email: info@saypro.online Call/WhatsApp: Use Chat Button 👇
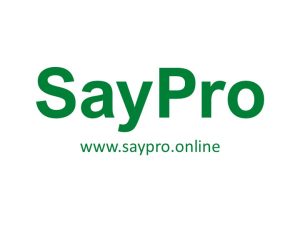
SayPro Data Analysis: Analyzing Quantitative and Qualitative Data
The Data Analysis phase of SayPro’s impact assessment is where the raw data collected through various methods is transformed into meaningful insights that address the key evaluation questions and shed light on the impact of SayPro’s interventions. This phase involves the systematic application of statistical tools for quantitative data and thematic analysis for qualitative data, followed by the integration of findings from both approaches.
Overarching Goals of the Data Analysis Phase:
- To systematically process and analyze the collected quantitative and qualitative data.
- To identify patterns, trends, and significant findings related to the impact of SayPro’s interventions.
- To answer the key evaluation questions developed during the planning phase.
- To generate evidence-based conclusions and recommendations for future SayPro activities.
Step 1: Data Preparation and Cleaning:
Before any analysis can begin, the collected data needs to be prepared and cleaned to ensure accuracy and consistency.
For Quantitative Data:
- Data Entry and Verification: Ensure that data from surveys, administrative records, or other sources is accurately entered into a suitable database or statistical software (e.g., SPSS, R, Python with Pandas). Implement double-checking procedures to minimize data entry errors.
- Data Cleaning:
- Identify and Handle Missing Values: Determine the extent and patterns of missing data. Decide on appropriate strategies for handling missing values (e.g., imputation, exclusion), justifying the chosen approach.
- Identify and Address Outliers: Detect and examine extreme values that may be errors or genuine but unusual cases. Decide whether to correct or exclude outliers based on clear criteria.
- Check for Inconsistencies and Errors: Review data for illogical entries, inconsistencies across variables, and violations of data integrity rules. Correct these errors or flag them for further investigation.
- Variable Transformation: Create new variables or transform existing ones as needed for analysis (e.g., calculating age from birth date, creating categorical variables from continuous ones).
- Data Organization: Structure the data in a way that facilitates analysis, ensuring clear variable labels and consistent coding.
For Qualitative Data:
- Transcription: Transcribe audio or video recordings of interviews and focus group discussions accurately and verbatim (or with appropriate levels of detail, depending on the analysis approach).
- Data Cleaning and Preparation:
- Review Transcripts for Accuracy: Check transcripts against the recordings for any errors or omissions.
- Anonymization and Confidentiality: Remove any identifying information to protect the privacy of participants. Assign codes or pseudonyms.
- Formatting: Standardize the format of transcripts and other qualitative data (e.g., field notes, documents) for easier analysis.
- Initial Reading and Immersion: Researchers should read through the qualitative data multiple times to gain a holistic understanding of the content and identify initial themes or patterns.
Step 2: Quantitative Data Analysis using Statistical Tools:
The choice of statistical tools will depend on the research questions, the type of data collected (e.g., nominal, ordinal, interval, ratio), and the study design.
- Descriptive Statistics:
- Calculate measures of central tendency (mean, median, mode) and dispersion (standard deviation, variance,1 range, interquartile range) to summarize the characteristics of the sample and key variables.
- Generate frequencies and percentages for categorical variables.
- Create visual representations of the data (e.g., histograms, bar charts, pie charts, box plots) to explore distributions and identify patterns.
- Inferential Statistics:
- Hypothesis Testing: Formulate null and alternative hypotheses to address the key evaluation questions. Use appropriate statistical tests to determine if there are statistically significant differences between groups or relationships between variables. Examples include:
- T-tests: To compare means of two groups (independent or paired samples).
- ANOVA (Analysis of Variance): To compare means of three or more groups.
- Chi-square tests: To examine associations between categorical variables.
- Correlation analysis: To assess the strength and direction of linear relationships between continuous variables (e.g., Pearson’s r, Spearman’s rho).
- Regression analysis (linear, logistic): To model the relationship between a dependent variable and one or more independent variables and to make predictions.
- Effect Size Calculation: Beyond statistical significance, calculate effect sizes to determine the practical significance or magnitude of the observed effects.
- Confidence Intervals: Estimate the range within which the true population parameter is likely to fall.
- Hypothesis Testing: Formulate null and alternative hypotheses to address the key evaluation questions. Use appropriate statistical tests to determine if there are statistically significant differences between groups or relationships between variables. Examples include:
- Longitudinal Data Analysis (if applicable):
- Use techniques such as repeated measures ANOVA, time series analysis, or growth curve modeling to analyze data collected over multiple time points and assess changes within individuals or groups.
- Statistical Software: Utilize statistical software packages (e.g., SPSS, R, Stata) to perform the analyses efficiently and accurately. Ensure that analysts are proficient in using the chosen software and interpreting the output.
Step 3: Qualitative Data Analysis using Thematic Analysis:
Thematic analysis is a widely used method for identifying, organizing, and reporting patterns (themes) within qualitative data. There are different approaches to thematic analysis (e.g., inductive vs. deductive, semantic vs. latent). SayPro should choose an approach that aligns with the research questions and the nature of the data. A common process involves the following steps:
- Familiarization: Immerse oneself in the data by reading and re-reading transcripts, field notes, or other qualitative materials to gain an overall sense of the content. Make initial notes and observations.
- Coding: Systematically identify and label segments of the data that are relevant to the research questions or are of interest. Codes can be descriptive (summarizing the content) or interpretive (reflecting the researcher’s understanding). This can be done manually or using qualitative data analysis software (QDAS) like NVivo, Atlas.ti, or MAXQDA.
- Searching for Themes: Group the initial codes into broader, overarching themes based on patterns of meaning, similarities, and interrelationships. This involves looking for recurring ideas, topics, and patterns of belief or behavior.
- Reviewing Themes: Refine the identified themes by checking them against the entire dataset to ensure they are well-supported by the data and accurately capture the essence of the coded segments. Some initial themes may be too broad, too narrow, or overlapping and need to be adjusted.
- Defining and Naming Themes: Clearly define the essence of each theme and develop a concise and informative name that captures its core meaning. Provide a detailed description of each theme, including illustrative quotes from the data.
- Producing the Report: Select compelling examples (quotes) from the data to illustrate each theme and present the findings in a clear and organized manner. Discuss the significance of the themes in relation to the research questions and the overall impact assessment.
Step 4: Integrating Quantitative and Qualitative Findings:
To provide a more comprehensive understanding of impact, it is crucial to integrate the findings from the quantitative and qualitative analyses. This can be done in several ways:
- Triangulation: Compare and contrast the findings from different data sources and methods to confirm, corroborate, or challenge the interpretations. Look for convergence and divergence in the results.
- Complementarity: Use qualitative data to elaborate on or provide context for quantitative findings (e.g., explaining the “why” behind statistical trends).
- Expansion: Use qualitative data to explore aspects of impact that were not captured by the quantitative measures, such as unintended consequences or changes in perceptions and experiences.
- Initiation: Use qualitative findings to generate new questions or hypotheses that can be further explored through quantitative analysis.
- Paradigm Mixing: Combine quantitative and qualitative approaches in a way that reflects different epistemological perspectives.
Step 5: Interpretation and Conclusion:
Based on the integrated analysis of quantitative and qualitative data, interpret the findings in relation to the key evaluation questions and the theory of change.
- Assess the Magnitude and Significance of Impact: Determine the extent to which SayPro’s interventions have achieved their intended outcomes and impacts, considering both statistical and practical significance.
- Identify Factors Influencing Impact: Explore which factors (e.g., participant characteristics, implementation quality, contextual factors) may have contributed to or hindered the observed impacts.
- Examine Unintended Consequences: Analyze any positive or negative unintended outcomes that emerged from the data.
- Draw Evidence-Based Conclusions: Formulate clear and concise conclusions that are directly supported by the data analysis.
- Acknowledge Limitations: Be transparent about the limitations of the data and the analysis methods used. Discuss potential biases and areas where further research may be needed.
Step 6: Documentation and Reporting:
Thoroughly document the data analysis process and present the findings in a clear, accessible, and engaging manner.
- Detailed Methodology Section: Describe the data analysis techniques used for both quantitative and qualitative data, justifying the choices made.
- Presentation of Findings: Use a combination of text, tables, graphs, and compelling quotes to present the key findings. Organize the findings in relation to the key evaluation questions.
- Integration of Findings: Clearly articulate how the quantitative and qualitative results were integrated to provide a holistic picture of impact.
- Discussion and Interpretation: Provide a thoughtful interpretation of the findings, linking them back to the theory of change and existing literature (if applicable).
- Conclusions and Recommendations: Based on the evidence, formulate clear and actionable recommendations for future SayPro activities, program improvements, and strategic directions.
- Appendices: Include relevant supporting materials such as data analysis code, interview protocols, coding frameworks, and detailed statistical outputs.
Software and Resources:
SayPro should consider utilizing appropriate software and resources for data analysis:
- Quantitative Data Analysis: SPSS, R, Stata, Python (with libraries like Pandas, NumPy, SciPy, Statsmodels).
- Qualitative Data Analysis: NVivo, Atlas.ti, MAXQDA, Quirkos, Dedoose (or even spreadsheet software like Excel for basic coding).
- Statistical and Qualitative Analysis Expertise: Ensure that the team conducting the analysis has the necessary skills and knowledge. Consider engaging external consultants if in-house expertise is limited.
By following a rigorous and systematic approach to data analysis, SayPro can generate credible and insightful findings that provide a strong evidence base for understanding its impact and informing its future work in Diepsloot. The integration of both quantitative and qualitative data will offer a richer and more nuanced understanding of the changes brought about by SayPro’s interventions.
Leave a Reply