Your cart is currently empty!
SayPro Prompts & Topic Extraction Analysis: Reviewing the effectiveness of the GPT topic extraction process and ensuring all prompts are optimized for maximum efficiency.
SayPro is a Global Solutions Provider working with Individuals, Governments, Corporate Businesses, Municipalities, International Institutions. SayPro works across various Industries, Sectors providing wide range of solutions.
Email: info@saypro.online Call/WhatsApp: Use Chat Button 👇
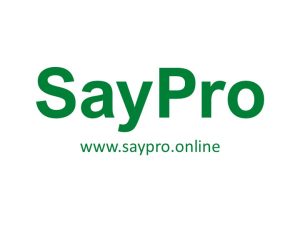
SayPro Prompts & Topic Extraction Analysis: Reviewing the Effectiveness of the GPT Topic Extraction Process and Ensuring All Prompts are Optimized for Maximum Efficiency
Introduction
The primary goal of the topic extraction process in GPT-based systems like SayPro is to efficiently identify relevant themes or subjects from a collection of textual data. This process is vital for a variety of applications, including summarization, content categorization, sentiment analysis, and more. Reviewing the effectiveness of this topic extraction process, and ensuring that all prompts are optimized for maximum efficiency, helps maintain high-quality results while reducing errors and inconsistencies. This analysis will discuss the effectiveness of GPT’s topic extraction process, the key areas where prompt optimization plays a crucial role, and how these factors contribute to improving the overall performance of SayPro prompts.
1. The Role of Topic Extraction in GPT Systems
Topic extraction refers to the ability of a language model to understand and identify the central ideas or themes from a given text. It involves several key sub-processes:
- Keyword Identification: Recognizing the most important terms and phrases within a body of text.
- Theme Classification: Grouping related keywords and concepts into overarching topics.
- Contextual Understanding: Ensuring that the topics identified are relevant in the context of the surrounding text.
- Hierarchy Creation: Organizing extracted topics into categories or sub-categories based on relevance or specificity.
For SayPro, the topic extraction process aims to produce accurate, relevant, and contextually appropriate topics that match user needs. In this process, GPT plays a crucial role by leveraging its vast understanding of language patterns and context to determine the most pertinent themes.
2. Reviewing the Effectiveness of the GPT Topic Extraction Process
Several factors determine how effective the GPT-based system is in extracting topics from a given prompt:
2.1 Precision and Recall of Extracted Topics
- Precision: The degree to which the identified topics truly represent the content of the text. For instance, if a text discusses global warming but the topics extracted are focused on unrelated themes (e.g., economic policies), this indicates a problem with precision.
- Recall: The ability of the system to identify all relevant topics in a given text. High recall would mean that most, if not all, important topics are captured without missing any significant ones.
Analysis: In GPT’s topic extraction, precision and recall depend on how well the model interprets the subject matter and organizes the extracted topics. Higher accuracy in context recognition would naturally enhance both precision and recall.
2.2 Contextual Relevance
One of the key advantages of GPT-based models is their ability to understand context. Contextual relevance ensures that the extracted topics are not only linguistically significant but also make sense in the broader framework of the discussion.
Analysis: A significant challenge lies in the model’s ability to correctly interpret multi-faceted discussions that may touch on multiple domains or sub-topics. Optimizing the prompts to give the model clear guidance about the context is essential for improving its understanding of the material.
2.3 Handling Ambiguity and Complexity
Texts with ambiguous terms or multiple meanings can lead to incorrect or incomplete topic extraction. For instance, the word “Apple” could refer to the fruit or the technology company. GPT’s ability to resolve such ambiguity is crucial for high-quality topic extraction.
Analysis: Ambiguity can be mitigated by designing prompts that provide sufficient context for disambiguation. Additionally, incorporating more specific keywords or references within the prompt can help reduce these errors.
3. Optimizing SayPro Prompts for Maximum Efficiency
To maximize the efficiency of GPT’s topic extraction, optimizing the prompts is key. Prompts need to be clear, specific, and structured in a way that directs the model toward identifying the correct themes. Several strategies can be employed to enhance prompt performance:
3.1 Use of Specific Instructions
Including detailed instructions within the prompt can guide the model toward more accurate topic extraction. For instance, instead of a vague prompt like “Extract topics from the following text,” a more specific prompt such as “Extract the main environmental and economic topics discussed in the following text” can significantly improve the relevance of the extracted topics.
3.2 Providing Contextual Information
When the topic of the text could fall into multiple domains, providing the model with some context or background information ensures that the correct topics are identified. For instance, when dealing with a technical article, specifying the industry or field (e.g., “Extract topics related to software development”) can help narrow down the possibilities.
3.3 Balanced Length of Input
While GPT is capable of handling large volumes of text, excessively long passages may cause the model to lose focus or dilute its attention to certain topics. On the other hand, overly short texts might not provide enough context for accurate topic extraction. Finding the optimal length for the input can improve topic identification.
3.4 Tailoring Prompts for Domain-Specific Language
For specialized domains (such as legal, medical, or scientific fields), creating domain-specific prompts ensures that GPT understands the context better. This can involve using industry-specific terminology and phrasing that aligns with the language of the target domain.
Example: If the goal is to extract topics related to climate change from a scientific paper, a prompt might read: “Extract the key environmental and policy topics discussed in this climate change study, including any references to carbon emissions, renewable energy, or governmental interventions.”
3.5 Iterative Prompt Refinement
Sometimes, the initial prompt may not generate the desired results, especially when working with complex or ambiguous texts. In such cases, iterating on the prompt by adjusting wording, adding more specific instructions, or breaking the text into smaller sections can help fine-tune the output.
4. Evaluating the Overall Efficiency of SayPro Prompts
To ensure that SayPro prompts are optimized for maximum efficiency, a feedback loop is essential. This involves:
- User Feedback: Collecting feedback from users on the relevance and accuracy of the extracted topics.
- Performance Metrics: Tracking key performance indicators (KPIs) like the number of relevant topics extracted, the time taken to process each request, and the accuracy of topics.
- A/B Testing: Experimenting with different prompt structures and analyzing the results to identify which versions yield the best outcomes.
Analysis: Continual monitoring and refining of prompts will allow for the incremental improvement of the topic extraction process, ensuring that the system is always evolving to meet user needs more effectively.
5. Challenges and Future Improvements
Despite the effectiveness of GPT in topic extraction, several challenges remain, including:
- Ambiguity in Complex Texts: Some texts are inherently complex and may require more advanced parsing techniques.
- Domain-Specific Knowledge: While GPT is proficient in many domains, more specialized knowledge (e.g., legal, medical) may need fine-tuning for optimal topic extraction.
- Contextual Understanding: In multi-topic or contradictory texts, maintaining high contextual accuracy is difficult.
To address these challenges, future improvements could include:
- Fine-tuning GPT on specific topics or industries to enhance topic extraction in those areas.
- Incorporating External Knowledge Bases to support the model’s understanding of specialized fields.
- Improved Ambiguity Detection to resolve conflicting topics within the text.
Conclusion
Reviewing the effectiveness of the GPT topic extraction process and optimizing SayPro prompts for maximum efficiency requires a balance of clear instructions, contextual understanding, and iterative refinement. By ensuring that prompts are well-structured, specific, and contextually relevant, GPT can produce highly accurate and useful topic extractions. Continuous improvements based on user feedback, performance analysis, and advancements in AI technology will help refine the process, ensuring that SayPro remains an effective tool for topic extraction in various contexts.
Leave a Reply